
Rakuten's India GCC moves beyond support, builds AI platforms for global Ops
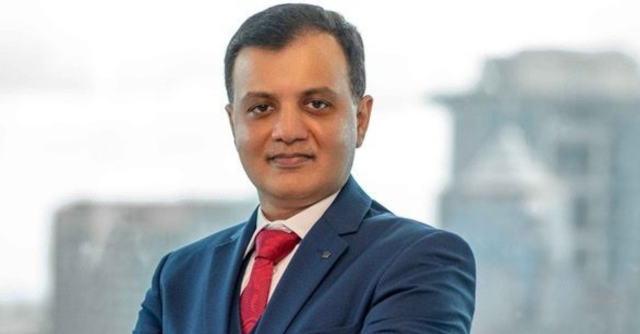

As Generative Artificial Intelligence (GenAI) and cloud technologies gain ground, Global Capability Centers (GCCs) in India are taking on more complex roles in enterprise tech.
Rakuten, with operations across e-commerce, fintech, and telecom, is using AI across core areas, productivity, business tools, customer experience, and operations.
In a conversation with TechCircle, Sunil Gopinath, CEO of Rakuten India, outlines how the company is scaling AI, the challenges of implementation in large, multilingual environments. The discussion also covers how GCCs are evolving and where Rakuten is focusing its tech investments next. Edited Excerpts:
With the rise of GenAI and other emerging technologies, where do you see enterprise technology heading? How is the landscape evolving?

We group our use of AI into four main areas. First is AI for productivity. This includes using AI to write and review code, helping engineers build better software. We've also applied it beyond engineering. Our HR and finance teams use LLM-based bots to answer employee questions about policies and benefits. Since these teams are small, the bots improve response time and efficiency by referencing uploaded documents.
Second is AI for business. This focuses on tools for merchants and content creators, like marketing automation, recommendations, search, and catalogue management. Many merchants upload basic product listings. We use GenAI to enhance these listings with better images, detailed descriptions, and pricing suggestions. These services improve visibility and performance, and merchants are willing to pay for them.
Third is AI for consumers. We use it in features like semantic search. Instead of searching for specific items, users can ask open-ended questions like, “What can I wear to a wedding?” The AI understands intent and suggests complete outfits, increasing relevance and boosting sales.

Fourth is AI for operations. Our SixthSense platform monitors all digital systems in real time, helping IT teams detect issues and trace root causes through causal graphs. It acts like a lightweight CIO, handling part of the operational workload.
What challenges have you faced integrating technologies like GenAI into enterprise systems, especially in a multi-market, multi-lingual environment like yours?
There are a few key things to consider when implementing AI. First, do you have the right data to make AI effective? AI is just a tool, it only works as well as the data it’s given. Its value depends on the quality, diversity, variety, and volume of that data. So, it’s important to make sure the data is available, clean, and properly labeled. In some organisations, this data exists and is usable. In others, it might be incomplete, messy, or not labeled correctly, which makes implementation harder.
Second, AI is non-deterministic by nature. It doesn’t always give a clear yes or no, or a perfect answer. You need to design systems that can handle this kind of uncertainty and work with outputs that may not always be exact. Third, training AI models takes time and resources. Even with good data, training requires a lot of effort and investment, especially in terms of computing power like GPUs, to get the model to perform well.

Fourth, privacy and data security are major concerns. AI systems can generate inaccurate or misleading responses, what we call hallucinations. There’s also a risk of employees unknowingly uploading sensitive company data to external AI services, like OpenAI, without realising it’s confidential. To prevent this, enterprise-grade AI systems need to have strong safeguards to protect company and customer data, and to keep AI outputs in check.
Finally, employee readiness is key. Many employees still aren’t fully comfortable using AI tools. Training is essential to help them get familiar with these tools and start using them effectively in their daily work. These are the core challenges that need to be addressed when scaling AI across an organisation.
How do you see GenAI reshaping GCCs in India, from both an industry and your company’s perspective? Do you think they’ll evolve into full-fledged product companies or continue as enablers for global enterprises
As I mentioned earlier, any large multinational organisation — ours or others, will face complex technology challenges. These can span consumer, enterprise, IT, and operations domains. There are hundreds of such problems being addressed at any given time.

Today, GCCs in India manage about 30–40% of a company’s technology operations. By nature, they are responsible for solving many of the critical business challenges. These span areas like commerce, financial services, supply chain, and crypto.
India has around a thousand GCCs, each working on different problem statements. GenAI has the potential to support nearly all of them. Use cases include advertising, creative content, pricing, fraud detection, and trading. GenAI will have an impact across nearly every domain.
Given this, most GCCs in India are expected to adopt GenAI to help solve large-scale technology problems for global companies.
Can you share how platforms like Rakuten SixthSense highlight India's growing ability to build enterprise-grade, AI-driven solutions?

This has been a valuable experience for us. We serve a diverse set of customers in India, including the Government of India, banks, retail companies, and healthcare organisations.
Many of our customers have been early adopters of observability tools, especially those powered by AI, commonly referred to as AIOps. The idea behind AIOps is that operations are increasingly being handled by AI rather than by adding more personnel.
These customers are open to considering solutions like ours that offer end-to-end observability. We collect and analyse data across the full stack: infrastructure, data centers, applications, databases, and user experience. When issues arise — whether at the business layer or in the user experience, we can trace the problem from start to finish to identify the root cause. This is powered by AI.

There’s strong interest in this because downtime or poor performance in digital applications has serious business consequences. For example, if a major bank's app is unavailable for 30 to 50 minutes, it can result in significant transaction losses. The same applies to large e-commerce platforms, if checkout, payment, or other key systems are down even briefly, they could lose hundreds of millions of dollars in revenue.
To prevent this, organisations are turning to AI-based observability tools like SixthSense. These tools monitor system health, identify what went wrong using causal graphs, and can also predict potential issues.
We've had customers using our platform for several years, and they continue to expand their use, adding more licenses and adopting additional solutions over time.
You've shared a lot about SixthSense already, but how does AI in this service go beyond traditional monitoring or predictive maintenance?
One of the core things we do is anomaly detection. Before a problem surfaces or an application fails, there are often early indicators at the hardware level, issues in load balancers, routers, memory, or storage. These systems generate logs and alerts that suggest something might be wrong, but they're often ignored because nothing critical has happened yet.
Over time, these ignored signals can escalate into major issues, like a database going down or a data center operating at reduced capacity. What we do is capture and analyse these alerts across various layers — hardware, supply chain, transaction systems, and connect them to identify early signs of failure.
This involves a detective-like process: taking seemingly unrelated pieces of information and piecing them together to predict an upcoming issue — or to understand what went wrong after an incident. Traditionally, this would require multiple engineering teams forming a war room and spending days or weeks tracking down the root cause. Our AI automates this process, acting like a digital detective to trace and correlate events in real time or after the fact.
Second, we go beyond application observability and also focus on data observability. That means monitoring your entire data infrastructure — pipelines, lakes, and systems, to ensure the data used for analytics is accurate, fresh, and trustworthy. Business leaders often make decisions based on dashboards, but if the underlying data is stale or corrupted, the insights are unreliable. Our system checks data quality, freshness, velocity, and lineage to validate whether analytics outputs can be trusted for decision-making.
Third, we also provide security observability. This includes monitoring not just applications buts APIs to detect misuse, unauthorised access, or data leaks. APIs connect internal systems with the outside world, and if left unmonitored, they can become a major vulnerability, allowing exposure of sensitive or personal data. Our platform watches this layer closely.
All of this is part of what we call SixthSense, which integrates these observability layers and is powered by AI. Another feature we’ve built is a conversational interface. For example, a CIO can ask, “What happened at 11 a.m. today?” and the system will investigate and respond with a summary. It might say that during a high-traffic event like Diwali, application volume spiked tenfold, overloading the system and causing the outage.
This removes the need for leadership to coordinate across multiple teams to diagnose the issue. Instead, they get immediate insights through a simple conversation with the platform.
As more companies adopt AI/ML, how do you see observability evolving in enterprise IT?
AIOps is set to become a core component of observability. The global observability market today is estimated at $10–15 billion and is projected to reach around $30 billion within the next five to six years. A significant portion of that growth will come from AIOps.
Traditional observability tools generate large volumes of alerts, notifications, and error messages. As systems grow more complex, engineers are overwhelmed by the volume of data. Without AI, it becomes difficult to identify what actually needs human attention. AIOps helps filter this information, surfacing only the most relevant issues.
Our approach involves end-to-end observability, not just the application layer, but also infrastructure, data, APIs, security, and user experience. This broad coverage enables a more complete understanding of what's happening across the system. It allows teams to trace issues from the user level all the way down to infrastructure, supporting more effective problem-solving.
This combination, AI-driven alert management and end-to-end observability, is central to how we see the industry evolving. It's also the foundation of our strategy.
Are there any other technologies Rakuten is investing in for the future?
At a broad level, there are two main areas of focus. The first is AI. The second is our cloud infrastructure. Rakuten Cloud consists of two parts. One serves enterprise needs and supports all 75 of our internal businesses, including e-commerce and fintech. The other is focused on telecom.
While I’m not directly involved in managing this area, Rakuten also operates a business called Rakuten Symphony, which provides telecom-as-a-service. This supports not only Rakuten Mobile but also third-party mobile operators. The offering covers everything end-to-end, BSS, OSS, antennas, base stations, all delivered via the cloud.
Unlike traditional telecom solutions that rely on hardware, Rakuten Symphony runs this infrastructure in software on our cloud. This telecom cloud is a major area of investment and represents the third pillar of our technology strategy.