
Vector database adoption in India set to surge, says TiDB’s Bhanu Jamwal
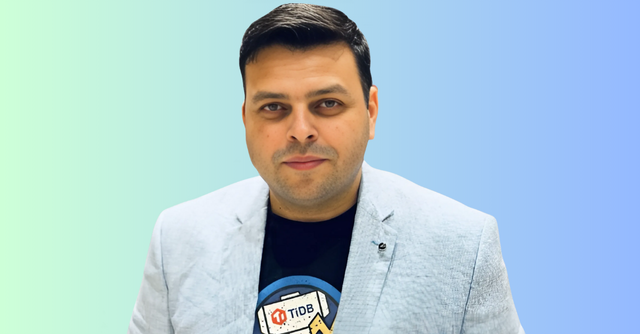

Vector databases are becoming crucial for Artificial Intelligence (AI) applications, especially with the rise of Generative AI and RAG. These databases store and process context-rich data, enabling more accurate AI insights. As AI adoption grows, businesses need scalable solutions for managing structured and unstructured data.
TiDB, founded in 2015, is a distributed SQL database adapting to the rise of vector databases in AI. In a conversation with TechCircle, Bhanu Jamwal, TiDB’s Head of Presales and Solution Engineering for APAC, shares how the company is responding to AI integration in India and the challenges enterprises face with vector adoption. Edited Excerpts:
How is vector database adoption evolving in India, and what are the key drivers behind this trend?

In the age of Generative AI, sparked by ChatGPT and large language models, innovation is booming. However, what really matters is making data relevant. This brings us to RAG (Retrieval-Augmented Generation), which ensures AI gives accurate, context-driven answers. The more context you provide, the more accurate the response.
As businesses test AI use cases, accuracy becomes crucial. AI cannot afford to provide inaccurate information, making context and RAG critical. This is where vectors come in. Vector databases, like Pinecone, store and process context-rich data efficiently. While vector databases aren't new, their adoption has surged, especially among AI companies.
However, not all businesses want a dedicated vector database, and some existing databases are now integrating vector support. The key is finding databases that can scale and handle growing volumes of vectorised data. The adoption of vector databases is just starting, and data storage on them will expand rapidly, up to 1000X. Ultimately, for AI to thrive, databases must support and scale vector storage effectively.
What do you think are the biggest challenges enterprises, especially in sectors like BFSI, face when integrating vector databases?

There are several challenges organisations face today as they adopt GenAI and work with Large Language Models (LLMs). Despite ChatGPT’s popularity over the past year, the underlying models are still very new, and organisations are experimenting with automation across their architectures.
One major challenge is scalability. As applications grow whether in OLTP systems, real-time analytics, or increased vector adoption organisations risk performance issues if their data platforms haven’t evolved. Traditional databases struggle to handle growing structured and unstructured data, leading to slow queries and latency. TiDB’s cloud-native, distributed SQL architecture enables businesses to scale AI workloads effortlessly without needing a complete re-architecture.
Another challenge is performance. Today, users expect instant results, as seen with AI models like GPT, which offer immediate responses. With traditional databases, performance often lags behind due to high data volumes. TiDB addresses this by providing a scalable solution that grows with your needs.

Additionally, modern applications require support for OLTP (transactional), real-time analytics, and AI workloads, which are typically siloed in different databases. TiDB combines all three into a single platform, simplifying integration and reducing the complexity of managing multiple systems. This HTAP architecture, Hybrid Transactional and Analytical Processing, ensures seamless operations and better security.
Lastly, managing multiple siloed databases leads to high costs. TiDB’s unified platform reduces overheads by consolidating OLTP, OLAP, and vector database functions into one. As an open-source solution, it also offers cost savings for organisations.
How does your company manage large volumes of structured and unstructured data in AI applications?
TiDB is a distributed SQL database, and it's important to understand how the database landscape evolved to appreciate its value. In the 90s, databases like MySQL, PostgreSQL, and SQL Server were single-node systems, great for handling fast transactions with low latency. But as data volumes grew in the 2000s with the rise of Web 2.0, these databases started facing challenges. Though some workarounds were applied, NoSQL databases emerged to address the need for scalable solutions with distributed architectures.

The problem with NoSQL was that they lacked support for standard SQL, which is the most widely used language by developers, offering ease of use, control over architecture, and data consistency. That’s where TiDB came in after 2015, combining the benefits of distributed systems with SQL’s familiar structure. TiDB scales effortlessly by adding nodes as your data grows, whether it's on virtual machines, Kubernetes, or cloud services, without any downtime.
With TiDB, applications scale in real time while maintaining high availability and consistency, ensuring that your data is always in good shape and compliant with ACID (Atomicity, Consistency, Isolation, Durability) principles. This makes TiDB an ideal solution for businesses facing rapidly growing data.
You mentioned HTAP earlier. Can you explain how its architecture benefits AI workflows?

HTAP (Hybrid Transactional and Analytical Processing) combines transactional and analytical processing into a single database. While it’s a complex architecture that few companies can master, TiDB has been designed to handle both workloads efficiently. With TiDB, you can run millions of transactions per second with low latency, while simultaneously performing real-time data analysis without impacting transactional performance.
This is crucial for organisations running AI models that require large amounts of data for accurate insights. For example, analysing just a week of data won’t provide strong insights, but with terabytes or even petabytes of data, AI models can generate much more accurate results. TiDB supports scalable data storage, enabling better accuracy without the need to move data across different platforms.
The benefits of this approach are clear. First, it provides access to larger datasets without the need to transfer data, which improves AI model accuracy. Second, it reduces costs by consolidating OLTP, OLAP, and vector databases into one platform.

Finally, it eliminates the challenges of data transfer, saving time, cutting costs, and minimising security risks associated with moving data. In summary, TiDB can handle vast volumes of data—from terabytes to petabytes, without complexity, making it an ideal solution for running AI models efficiently and accurately.
Given the growing integration of AI in enterprise solutions, how do you see the role of vector databases evolving in the next 3-5 years, particularly in India? What steps is your company taking to stay ahead in this changing landscape?
Vector adoption is growing rapidly, especially in the context of RAGs (retrieval-augmented generation). While not all data is stored in vectors yet, more systems are adopting them, with the primary driver being RAGs. These come in different architectures like Naive RAG, MultiModal RAG, Agentic RAG, and Hybrid RAG, but they all share a common reliance on vector databases. While some use graph databases, most leverage vectors for efficiency.
Innovation in the vector space is happening fast, particularly around improving data access and storage performance. In the next few years, we’ll see even more developments. Companies like Pinecone are already making strides, but these solutions require additional infrastructure.
TiDB's vision is to innovate on our existing data models. We already support OLTP and real-time analytics for over 3,000 customers, but we aim to integrate vector features and capabilities common in NoSQL databases, such as full-text search and advanced indexing. Our goal is to combine relational, OLTP, OLAP, and vector AI use cases into one platform, also tackling NoSQL challenges. With support for JSON and JSON indexing, TiDB will soon offer the advanced capabilities of NoSQL databases while maintaining high performance.