
Firms move to small language models to cut costs, gain efficiency and customisability
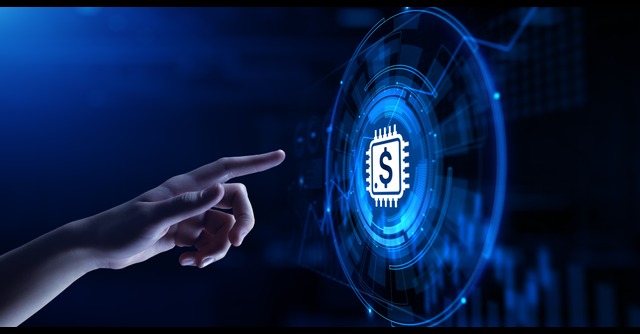

Llama, Phi, Mistral, and Granite may sound like characters straight out of a fantasy story, but small language models (SLMs) are becoming increasingly important in the AI landscape. While large language models (LLMs) have garnered much attention, companies are recognising the strategic benefits of SLMs for targeted, efficient, and cost-effective AI solutions.
SLMs are computational models designed to respond to and generate natural language. They are trained for specific tasks while using fewer resources than larger models. Global companies like Google, Microsoft, Samsung, Apple, Mistral AI, and Cohere, along with Indian IT firms such as Infosys, Tech Mahindra, and HCLTech, are actively developing SLMs tailored for various industries.
An edge over LLMs

Experts point out that LLMs, which power AI chatbots like OpenAI’s ChatGPT and Google’s Bard, have significant drawbacks that hinder technology adoption. Indian tech firms are focusing on SLMs to reduce costs and customise services for sectors like banking, cybersecurity, and telecom. Kunal Purohit, President of Next-Gen Services at Tech Mahindra, believes SLMs will revolutionise AI solutions by enhancing efficiency and accelerating integration, encouraging enterprises to invest in optimising their inferencing capabilities.
A major advantage of SLMs is their cost-effectiveness, they provide comparable performance to LLMs for specific tasks at lower costs, making AI more accessible to small and medium-sized businesses. Purohit noted that SLMs also enable real-time on-device processing, which is ideal for managing sensitive data without internet connectivity.
Balakrishna D. R., Executive VP at Infosys, added that SLMs often achieve higher accuracy and better domain adaptation than LLMs in resource-constrained environments, addressing data security concerns in regulated industries.

LLMs such as GPT-4, and SLMs can understand and generate natural language but are optimised for specific tasks. They are trained on focused datasets, excelling in tasks like customer feedback analysis and generating product descriptions. SLMs have significantly fewer parameters than LLMs, enhancing speed and efficiency. That said, while LLMs like GPT-4 have over 175 billion parameters, SLMs typically range from tens of millions to under 30 billion. This streamlined architecture allows SLMs to perform natural language processing tasks in specific domains with much less computational power, making SLMs suitable for resource-constrained environments like edge devices and mobile applications.
Ganesh Gopalan, Co-Founder and CEO of Gnani.ai, a conversational AI platform for enterprises, emphasised that SLMs' ability to operate on edge devices enhances accessibility and efficiency, improving data privacy through on-premise or offline processing. SLMs can be fine-tuned for specific domains, increasing accuracy in niche applications like finance, healthcare, and customer service.
Avirag Jain, CTO and Executive VP at R Systems noted a trend where companies frequently begin with large general-purpose models to explore various use cases, only to find the compute costs unsustainable. “As generative AI becomes more prevalent in enterprises, companies will likely leverage smaller models, fine-tuning them with proprietary data to achieve desired performance at a fraction of the cost,” he said.

Additionally, SLMs' targeted nature enhances accuracy while addressing data privacy and control concerns, allowing better data management and reducing potential copyright issues associated with LLMs. Geeta Gurnani, IBM Technology CTO & Technical Sales Leader, India & South Asia, further noted that in addition to increased efficiency and lower costs, SLMs are environmentally sustainable due to their lower energy consumption.
However, SLMs have limitations. Ram Kumar, Chief Technology Officer at Phi Commerce, pointed out that these models may struggle with nuanced language comprehension, contextual subtleties, and complex tasks due to their training on smaller specialised datasets. Despite these challenges, Kumar believes SLMs signify a shift in enterprise AI strategies from experimental to strategic, purpose-driven implementations that are more focused and cost-effective.
Is the future hybrid?

The future of AI may lie in hybrid models that integrate SLMs and LLMs for efficiency and versatility. Purohit anticipates that combining LLMs and SLMs will shape AI's future, creating efficient, scalable systems that address cost, privacy, and resource concerns. Jain explained that hybrid architectures could intelligently assign tasks based on complexity, with SLM managing simpler queries on edge devices and LLMs tackling more complex challenges.
The recent DeepSeek model exemplifies a hybrid approach for that matter. Although it is classified as an LLM due to its capabilities, it prioritises efficiency and can operate on lower-powered hardware. DeepSeek employs distillation techniques to create smaller, more accessible versions of its models, making them suitable for resource-constrained environments.
GlobalData forecasts that the overall AI market will reach $909 billion by 2030, with a compound annual growth rate (CAGR) of 35% from 2022 to 2030. In generative AI, revenues are expected to grow from $1.8 billion in 2022 to $33 billion in 2027, driven by the adoption of specialised custom models. By minimising hallucinations and ensuring data security, SLMs offer reliable solutions for enterprises across various sectors.

Going forward, the future of AI is likely to combine the strengths of both large and small models—large models offer broad capabilities, while small models provide efficiency and adaptability for specific tasks. Gurnani said, that business leaders must choose the right model based on the use case they are trying to solve that would maximise RoI and optimise resource use, ultimately ensuring responsible development and deployment of AI.