
Measuring the Impact of Generative AI in IT Services
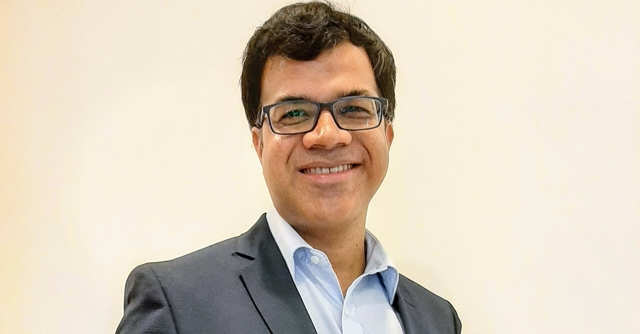

Generative AI is one of the most talked topics today in IT services. It refers to AI advancements that create new content in the form of requirements, designs, code, test cases or solution outlines through pattern recognition from past data.
People have both excitement about the potential productivity boost from Gen AI assisting humans in various ways, as well as fear from the likely reduction in certain job opportunities with Gen AI taking over some of the work which was performed by humans till now. We also read news of various leading technology companies investing billions of dollars into both the development and the adoption of Gen AI in IT services. The challenge lies in measuring the actual impact and aligning investment to maximize return on investment (ROI).
Key Areas and Metrics

The Impact of Gen AI can be viewed in four key categories – better, faster, cheaper and newer.
Better here refers to Gen AI's ability to assist in improving requirements, design, coding, and testing. Metrics like requirements completeness, technical debt score, code review depth, maintainable code, bug rate, test coverage, team satisfaction, and customer satisfaction can help assess its impact.
Faster emphasizes how Gen AI helps humans complete tasks more quickly. This can be measured through metrics such as developer velocity, median lead time for changes, deployment frequency, build duration, and issue resolution time.

Cheaper highlights Gen AI's promise to automate tasks, reducing manual labor and associated costs. Key metrics to gauge this include cost per deployment, value delivered per headcount, infrastructure cost savings, and resource allocation efficiency.
Finally, newer focuses on innovation, with Gen AI enabling rapid prototyping and personalized solutions. Innovation can be measured by the number of Gen AI-powered solutions, revenue from new solutions, win rates, and market leadership indicators. An alternative way of measuring this impact would be to classify the above metrics into three categories – operational, financial and experience metrics.
Process and Tools

Knowing what to measure is good start, but the next step is determining how to measure it. Firstly, we should establish pre-implementation baselines for the metrics. Next, measure the metrics during implementation at periodic intervals, say weekly. Finally, asses the post-implementation outcomes to ensure impact of Gen AI is quantifiable and actionable. We can leverage various tools across different aspects of development and project management to enhance efficiency and assess performance.
For DevOps and project management, tools like JIRA, Devlake, and Azure DevOps help track task completion rates, deployment frequency, and project efficiency. In terms of Coding Assistants, tools such as CodeRabbit and Tabnine allow us to measure the contribution of AI-generated code, coding speed, and defect rates. To ensure code quality analysis, tools like GitHub and SonarQube can evaluate the quality and maintainability of code. For performance monitoring, tools like Datadog and New Relic can help assess the reliability and scalability of AI-integrated systems in production. In model lifecycle management, tools such as MLflow and Kubeflow monitor the training, testing, and deployment of AI models, helping gauge ongoing performance improvements. Finally, survey and feedback tools like Qualtrics and Google Forms enable us to collect qualitative data on developer and client satisfaction, providing insights into user experiences.
Challenges to Overcome

Even after deciding on the key areas, metrics, process and tools to measure the impact of Gen AI, there will be some practical challenges to overcome.
Resistance to change will perhaps be the biggest challenge, as multiple stakeholders including members of the IT team, managerial staff, business users and financiers’ needs and concerns must be addressed. Another challenge lies in measuring non-financial benefits. While we discussed various metrics to measure impact, the challenge will be do a holistic cost-benefit analysis considering non-financial benefits in addition to financial benefits and costs. Lastly the integration issues present a challenge in ensuring that Gen AI tools and measurement tools seamlessly integrate into the existing development lifecycle, while maintaining security and compliance considerations.
A well-planned Gen AI adoption roadmap with continuous monitoring, stakeholder involvement and regular feedback loops should be able to address these challenges.

Let me conclude by saying that to capture the immense potential value of Gen AI in IT services, we need to follow a structured approach to measure Gen AI's impact. This will require action for IT leaders to adopt measurement frameworks to enhance decision-making and find practical solutions to challenges as they do that.
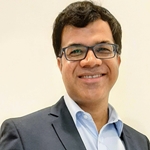
Soumya Ranjan Dash
Soumya Ranjan Dash is Operating Principal of Ezingocer India.