
GenAI’s shift from text to multimodal capabilities unlocking new industrial use case: Happiest Minds exec
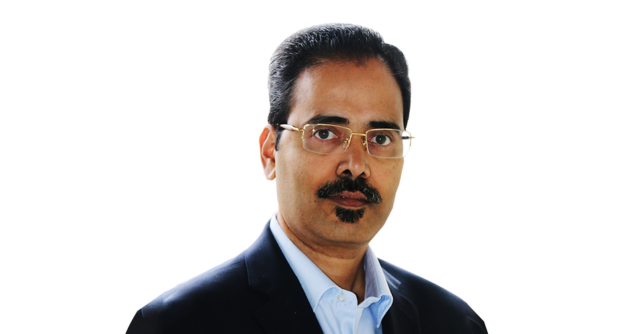

Happiest Minds, an IT services providers, has been using Generative Artificial Intelligence (GenAI) to support customer growth and success. In its latest financial report, the company reported strong growth, driven by the integration of such technologies. The company has also established a dedicated business unit for GenAI to focus on latest opportunities.
In a conversation with TechCircle, Sridhar Mantha, CEO of the GenAI Business Unit, shared his views on the current AI landscape. He discussed the impact of GenAI, especially after the release of ChatGPT, and addressed challenges like data scarcity in India, the importance of ethical AI, and the potential of technologies like quantum computing and edge AI. Edited excerpts:
How do you see evolving GenAI landscape, and as per you, what is the most significant trend shaping the industry today?
At Happiest Minds, we have always focused on leveraging digital technologies to drive customer transformation. For over three years, we’ve actively explored GenAI, starting with early models like BERT. Initially, these efforts were limited to small projects and proofs of concept.

The release of ChatGPT by OpenAI marked a turning point, akin to the iPhone revolution for smartphones. Its powerful model and widespread adoption, quickly reaching 100 million users, spurred significant customer interest, particularly in the EdTech sector. Recognising GenAI’s transformative potential, our board and management made it a cornerstone of our growth strategy.
Unlike many others, we established a dedicated business unit for GenAI, with a complete team structure. As Group CTO and now CEO of this unit, I’ve overseen its formation and growth over the past year.
GenAI's impact has been profound across industries, with early adoption concentrated in EdTech due to its text-heavy use cases like virtual tutors. The technology’s influence has since expanded rapidly across sectors.

Looking ahead, forecasts indicate GenAI will elevate the AI market by adding a powerful new layer to proven AI solutions. However, adoption will follow a typical curve — early movers will lead, while others may take 6–18 months to assess return of investment (ROI). Despite this, we expect a steep acceleration once the benefits are established.
Which industries or verticals, in your opinion, stand to benefit the most from this technology?
This technology, is a strong horizontal solution compared to narrower technologies like Internet of Things (IoT), which have a more focused appeal, such as in manufacturing or industrial sectors. Similar to broader AI, GenAI is gaining traction across all verticals we’re working with. The pace of adoption depends on who acts more aggressively within a three- to six-month window.
Initially, we saw interest from the education sector, but traction quickly grew in retail due to its customer engagement, support, and other use cases. Manufacturing and healthcare are also seeing significant uptake. While the technology is largely omnipresent, adoption in some sectors, like banking, financial services and insurance (BFSI), is slower due to concerns around regulations, particularly in banking. Overall, the excitement and adoption are highly horizontal.
What common challenges do your clients face when integrating GenAI, and how do you help them overcome these obstacles?

Regulations aside, governments are moving quickly, but let’s focus on the technology itself, particularly in manufacturing. GenAI is advancing rapidly. Initially, many problems could be addressed with text-based solutions. However, the shift toward multimodal AI interpreting images and processing large PDF content has unlocked entirely new possibilities for industrial applications.
For example, once models gained the ability to understand images, we saw a surge in innovative use cases among industrial customers, like analysing shop floor nameplates. This progression marks a clear trajectory: from text-based AI to multimodal capabilities, and now toward logical reasoning and decision-making, which we’ve started observing in recent months.
Each advancement text, multimodal, reasoning, represents a new wave, expanding use cases and driving excitement for implementation across industries.
What are your thoughts on ethical AI usage, and how is your company ensuring responsible AI practices in its solutions?

This is a multilayered challenge requiring robust governance at every level to ensure the ethical use of complex technologies. At the foundational level, the underlying models must adhere to stringent ethical standards — a focus for companies like Microsoft, Amazon, and OpenAI. Despite this, occasional failures, such as bias or over-corrections, still occur even among major players.
At higher levels, additional guardrails are necessary to ensure solutions remain aligned with ethical standards and specific use cases, even if issues arise in the base models. Our AI governance framework, part of our cybersecurity and ethics practices, is integral to our solution development.
During design, implementation, and testing, we address functionality, quality, cybersecurity, and ethics. This includes detecting bias, aligning with governmental standards (e.g., the European AI Act), and applying guardrails to prevent ethical violations or biases from manifesting in our solutions.
With GenAI, there are always concerns about data, including data scarcity, especially in India compared to the West. How do you approach this challenge?

In my opinion, building a powerful model depends on two key factors: the algorithm and base model, and the quality and volume of training data. Even with an excellent model, inadequate or poor training data limits its learning capabilities.
In the West, most training data, such as content from Reddit, Quora, and other online sources, is already available. This creates a saturation point for model evolution since the quantity of new training data is limited. For example, as models like GPT progress to versions like 5.0, improvements are constrained if the training data remains largely unchanged.
In the Indian context, the availability of digitised information in regional languages like Telugu, Hindi, Tamil, or Bangla is limited, despite ongoing efforts to digitise published works. Currently, models are capable of casual conversations in multiple languages. For instance, we've seen success in projects where policy documents in any language can be queried in Indian languages like Kannada or Hindi, providing conversational responses.

However, as models advance into reasoning or more complex tasks, these limitations become more evident. That said, initiatives by the Indian government and NGOs to digitise historical documents and literature are steadily increasing the availability of training data.
Today, if you ask ChatGPT a question in Telugu, it can provide a reasonable conversational answer. However, when it comes to niche areas like poetry in Hindi or Tamil, its limitations are apparent due to a lack of relevant training data. These gaps will diminish as more digitised content becomes accessible.
How do you plan to integrate upcoming trends or other technologies like quantum computing, multi-modal AI or edge AI into your offerings?
Quantum computing has made significant strides, especially with hyperscalers like AWS and Azure offering quantum computers on a pay-as-you-go model, similar to traditional servers. This accessibility has opened new opportunities, but its applications remain niche. One promising area is quantum machine learning, where specific machine learning models benefit from quantum infrastructure. Currently, we have a small team working on a healthcare project to explore such possibilities. However, widespread adoption remains a few years away, likely limited to specialised algorithms optimised for quantum systems.
On another front, edge computing is gaining momentum, especially in AI. With IoT data generation increasing latency when processed via the cloud, edge computing offers a practical solution. Advances in AI model efficiency are driving this shift. For instance, large models with 175 billion parameters initially required extensive cloud infrastructure, but smaller models (1.5–7 billion parameters) now perform well on edge devices. Mobile phones are a key example, with companies like Apple and Android embedding GenAI capabilities directly into devices. This reduces latency and enables efficient tasks like voice-to-text and simple predictions at the edge, paving the way for broader adoption in other compact devices with small yet powerful language models (SLMs).
What is your company’s approach to building sustainable AI systems, given the environmental concerns related to training large AI models?
You're absolutely right that large models, especially in their initial stages, require extensive compute infrastructure for both training and inference. However, we’re now seeing a shift toward smaller models, which naturally reduces the strain on compute resources.
When dealing with massive models like those with 300 billion parameters, the demands on data centers such as electricity and cooling are significant. However, as workloads increasingly shift to the edge and standard compute infrastructure, the reliance on large models will decrease. This challenges the assumption that all models must be enormous and resource intensive.
We focus on smaller, edge-deployed models rather than exclusively on mega-models. This approach is more cost-effective, responsive to customers, and environmentally friendly. By utilising models like Microsoft Phi, with just a few billion parameters, we can achieve similar results with much less computational demand compared to mega-models like those used in cloud or ChatGPT.
Are there any new services or offerings related to GenAI that you're coming up with next?
We’ve established a GenAI business services unit focused on developing solutions for customer-specific use cases. Given the rapid pace of research and evolution in this field, our offerings have quickly expanded. Initially, we concentrated on text-based use cases but recognising that enterprises rely on more than just text, we moved into data-driven solutions.
With the emergence of multimodal Large Language Models (LLMs), we developed applications involving images. Recognising the demand for more interactive solutions, we introduced text-to-voice and voice-to-text capabilities, followed by innovations like avatar-based kiosks that engage users conversationally.
Looking ahead, we’re focusing on reasoning capabilities, such as agentic architectures with multiple collaborating agents, moving beyond monolithic LLM-based solutions. We are exploring use cases involving logical deduction and reasoning.
Our internal R&D teams, in collaboration with Microsoft and as part of their AI Partner Council, gain early insights into emerging technologies. This enables us to continuously enhance our solutions by integrating GenAI with broader AI and automation to address increasingly complex challenges.