
Snowflake's Prasanna Krishnan on tackling GenAI growth with security and compliance
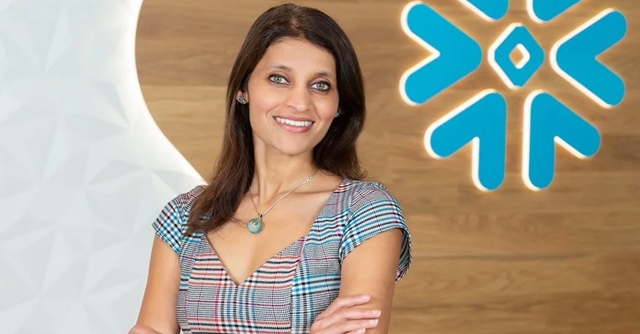

The Generative Artificial Intelligence (GenAI market will grow from $11.3 billion in 2023 to $51.8 billion in 2028, as per an August 2023 report by MarketsandMarkets, reflecting a compound annual growth rate (CAGR) of 35.6%. As this sector expands rapidly, organisations must prioritise understanding the data governance and security commitments that come with integrated AI services.
In a conversation with TechCircle, Prasanna Krishnan, Head of Collaboration and Horizon, Snowflake, shed light on the evolving compliance and security challenges enterprises encounter when adopting AI solutions. She also discussed Snowflake’s strategic focus for the future. Edited Excerpts:
How does Snowflake address the evolving compliance and security challenges that enterprises face when using AI Data Cloud solutions?

Compliance is a core pillar of Horizon Catalog, covering several important aspects. First, it involves understanding how data is used, identifying and classifying sensitive data, and then protecting it. This is a key step toward ensuring compliance. As data access is democratised, it's critical to adhere to regulations, especially when handling sensitive information like personally identifiable data.
Automating data classification, applying policies, and ensuring governance while still allowing access to insights from sensitive data is crucial. Our comprehensive compliance toolkit includes features like automatic data classification and data protection policies, including clean rooms for secure data collaboration.
Snowflake also meets high-security standards, supporting government regions and certifications like FedRAMP in the US, which are part of the compliance measures offered through the Snowflake AI Data Cloud.

Can you elaborate on how Snowflake ensures data privacy and security, especially for enterprises handling sensitive information in industries like healthcare and finance?
Snowflake has prioritised security and privacy from the start, with tailored protections for industries like financial services and healthcare. All data is encrypted at rest and in transit, forming the foundation of Snowflake's approach to data security. Beyond encryption, protecting sensitive data is critical.
In healthcare, for example, patient data is highly sensitive and remains fully under the customer’s control by default. If teams within an organisation need insights from this data without exposing it, policies like data masking can be applied. This allows users to query data without viewing sensitive information. More advanced methods, like differential privacy, enable insights without revealing the underlying data, with only a few privileged users having access to raw information.

Additionally, Snowflake's clean rooms add another layer of security. If a query could identify an individual based on certain factors, no results are returned if the number of rows is below a set threshold, ensuring privacy while still providing valuable analytics.
With the rapid adoption of GenAI, what specific security measures does your company implement to safeguard intellectual property and prevent data breaches?
Let's address both aspects of GenAI. With its rapid adoption, the need for governance and protection has grown. Snowflake is committed to making enterprise AI both efficient and secure. A key element is enabling customers to use AI models with their own data, while ensuring they retain full ownership. The model runs entirely within the customer's account, and we do not access or use their data to improve the model or share it across other customers.

Through Snowflake Cortex, customers have complete control over their data. We offer pre-built Large Language Models (LLMs) that can be fine-tuned with the customer's own data, all within the security boundaries of their account. No data leaves the customer’s environment. This approach ensures AI models are applied to the data, rather than moving the data, preserving governance and security throughout.
What are your thoughts on the data scarcity challenge in India compared to the West, especially regarding GenAI and LLMs?
GenAI thrives on data, the more you have, the better your models will perform. However, in regions like India or specific industries with limited data, sharing data in a privacy-compliant way becomes incredibly valuable. This is where Snowflake's AI Data Cloud excels.

For example, imagine a group of hospitals, each with its own data. By sharing their data, they could gain far deeper insights, but they must do so while protecting personal information. Snowflake's data-sharing capabilities, using features like a clean room, enable secure, compliant collaboration, helping organisations overcome data limitations while maintaining governance.
What’s the biggest challenge enterprises face when implementing AI solutions, and how does your company help them overcome it?
The first key to a successful AI strategy is having a strong data foundation. It's crucial to centralise your data and break down silos while ensuring proper access controls are in place. This foundation is essential for unlocking AI's full potential, and it's where Snowflake shines. For example, Fidelity Investments in the US shared at our conference how they built a unified data platform on Snowflake, enabling teams to effectively leverage AI and models.

We help businesses create this solid foundation by integrating data from different sources and eliminating silos. Another major challenge is securing and governing data access, which is critical for enterprise AI. Snowflake addresses this with our Horizon Catalog, offering robust security, compliance, and privacy features to protect and audit AI-driven data.
Are there any specific trends you’re seeing in the market regarding how enterprises are redefining their data strategies to stay competitive in AI technology?
A growing trend we're seeing is companies recognising the need to break down data silos. In the past, data was scattered across various databases and systems — some on-premise, some in the cloud — while enterprise applications like customer relationship management (CRM) and supply chain systems kept their data isolated. Now, businesses are shifting towards consolidating this data in Snowflake as a single source of truth, which supports the development of LLMs and applications built on that data.
This involves migrating data into Snowflake or, for those using open table formats like Iceberg, Snowflake can support that as well. Snowflake allows data to remain in cloud storage in an Iceberg-compatible format, representing it as Iceberg tables within Snowflake. These tables can then leverage Snowflake’s security and privacy features. Additionally, Snowflake’s native app-based connectors allow data from various enterprise systems to be brought into a customer’s account, effectively breaking down silos.
This approach enables companies to build a strong data foundation on Snowflake, consolidate their data, set access policies, and ensure effective data protection.
How do Snowflake Native Apps enable cross-industry collaboration, and what specific benefits do they bring to organisations looking to enhance their data usage?
Traditionally, accessing data for enterprise applications was a long and complex process. You’d have to set up EC2 instances, deploy the application, and configure multiple settings to connect to a database. This often resulted in creating duplicate data across applications, leading to governance issues.
Snowflake's native applications simplify this by providing seamless access to data through a single source of truth with built-in governance. Think of it like installing an app on your phone — you go to the app store, find what you need, and install it with a few clicks, granting access as required.
With the Snowflake native app framework, this ease of use is now available for enterprise applications. These native apps come packaged with logic, and sometimes data, that you can deploy directly in your Snowflake account, keeping everything under your governance.
For example, you can browse the Snowflake Marketplace (similar to an app store) for an identity resolution app. Once enabled, the app runs in your account with permissions you define, like writing to specific tables or running background tasks.
The power of these applications lies in their ability to operate within the customer’s account based on granted permissions. They can securely access data from the provider without exposing that data to the customer. For instance, a native app can enrich contacts within your account without giving you direct access to the provider’s data.
This approach eliminates the need to move data or create copies, allowing applications to run within a secure environment while ensuring providers retain full control over their application logic and intellectual property.
How do you see Snowflake Natives apps as a key driver for the future of AI driven data in collaboration?
Native applications let you package data, logic, and models for easy distribution on Snowflake, making it simpler for consumer accounts to access and use them. This setup is especially valuable for building and sharing generative AI applications.
Many apps on Snowflake already use this capability. For instance, you could create a native app with a function to calculate fraud scores, using AI to run complex models while protecting the provider's intellectual property. The app can then be distributed through the Snowflake Marketplace or privately.
Consumers can run the fraud score function directly in their account without moving any data, ensuring security. This approach allows AI application providers to distribute their solutions via Snowflake’s network, and consumers can seamlessly apply them to their data in a trusted, governed environment. Additionally, consumers can use Snowflake’s marketplace payment methods, including monetisation options, to pay for these applications.
Looking ahead, are there any other technologies that Snowflake is focusing on besides generative AI?
GenAI is a key pillar of our workloads, but our broader AI data cloud vision revolves around a unified platform where all your data resides. This single platform enables various personas — whether they're data engineers, analysts, scientists, or business users — to work seamlessly with one source of truth.
GenAI is one task among many. For example, a developer or data scientist might integrate generative AI into an application that a business user then accesses. But Snowflake is also innovating across other critical areas of the data journey. We’re enhancing data pipelines, making it easier for data engineers to build them declaratively with dynamic tables.
We're also investing in advanced analytics, supporting geospatial and time-based data analysis, and expanding Snowpark to allow programming in any language. Snowpark Container Services further simplifies managing containerised applications on Snowflake. In short, our focus spans multiple pillars: generative AI, data engineering, advanced analytics, pipelines, and applications.