
Why India needs to build more indigenous LLMs
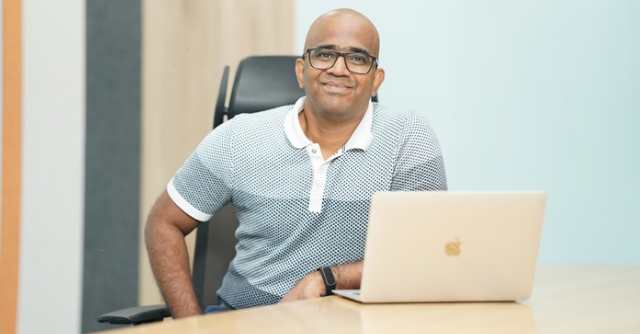

Large Language Models such as GPT, Gemini, and Llama have brought about a seismic shift in the AI (Artificial Intelligence) landscape, transforming how we interact with technology. Trained on massive amounts of data, these models are at the heart of the generative AI boom, excelling in tasks such as translation, content creation, and even answering questions in a way that feels almost human. Their ability to process language naturally makes them an invaluable tool for businesses looking to drive efficiency and engagement at scale. However, as powerful as they are, Large Language Models also raise serious concerns about privacy and data security.
Privacy, Bias & Compliance: Key Challenges Posed by Offshore LLMs
So far, we have relied on LLMs developed and maintained by companies, often located in other countries. While these models offer cutting-edge capabilities, they provide limited visibility into how their data is managed or processed, or whether they meet regulatory standards. This becomes even more crucial when processing requests that contain sensitive or confidential information, such as healthcare and financial data, where privacy is a top priority. There is an added risk of AI bias, which can originate from the perspectives of data collectors, inherent biases in the data, or the sources used for training the models. If an LLM is trained in disinformation, it can rapidly generate highly convincing false information, backed by strong rhetorical skills. Moreover, there is a lack of clarity over copyright infringement and ownership of AI-generated works. With offshore models, there is limited recourse to address these issues effectively. AI systems can malfunction when exposed to untrustworthy data, and there is no foolproof defense against cyberattacks. These issues can be in direct conflict with national data sovereignty principles, leading to legal and compliance challenges. India's Digital Personal Data Protection (DPDP) Act allows users the right to be forgotten and to have their data deleted. However, when user data is used to train an LLM, the individual pieces of data become interwoven into the complex model itself. This makes it technically challenging, if not impossible, to erase the data. Mitigating these risks involves a multi-faceted approach, including using diverse and unbiased training data, ensuring transparency in data handling, and implementing robust privacy measures tailored to local regulations. This is where indigenous LLMs come in.

Why Indigenous LLM Matters
Indigenous LLMs are homegrown solutions developed within a specific region and trained on data reflecting local culture, language, and privacy regulations, mitigating the risks associated with outsourcing. Here’s why developing iIndigenous LLMs is crucial for security as well as long-term growth:
Data Sovereignty: Indigenous LLMs keep data local, reducing the risk of it being accessed by entities outside your region. This helps avoid legal issues and builds trust among stakeholders. Moreover, stakeholders will have greater confidence in the organisation’s commitment to protecting their information.

Enhanced Security: When LLMs are developed locally, they can be tailored to align with the specific security protocols and privacy regulations of the region. This means that these models can incorporate advanced security features from the outset, significantly reducing the likelihood of unauthorised access or data breaches.
Greater Control and Transparency: With indigenous LLMs, organisations have complete oversight of how their data is managed and protected. This level of control is vital for maintaining data security practices and reassures users that their information is being treated with the utmost care.
Customisation and Optimisation: The development of indigenous LLMs offers the flexibility to create solutions that are precisely tailored to meet the unique needs of an organisation. This could involve optimising the model to understand industry-specific use cases or to comply with security requirements.

Culturally Nuanced Applications: Indigenous LLMs have the unique advantage of being trained on datasets that reflect local culture, language, and societal norms. This localized training allows LLMs to learn from relevant data, enhancing their contextual understanding.
Why India Needs to Build More Indigenous LLMs
Currently, most LLMs only understand English. Using them for regional languages, especially in business, is tricky because they often make mistakes. We should not expect offshore LLM developers to address these problems because at their scale it might not be financially beneficial for them. In fact, private models work more accurately and faster than LLMs trained on platforms like ChatGPT or others. Building quality datasets remains the most challenging task for those wanting to build Indic LLMs. It involves a huge collaborative effort, including digitisation of books, working with linguists, engaging communities, and organising large-scale content creation workshops. It may be challenging, but it is not impossible. In fact, Ozonetel has been involved in a series of initiatives to create an Open AI ecosystem where indigenous LLMs are developed and utilised. Last year, we partnered with Swecha (NGO) to build a Telugu LLM called AI Chandamama Kathalu, a 7-billion-parameter open-source 'story' dataset trained on 40,000-45,000 pages of Telugu folk tales. This will be integrated with other business solutions to capture the pulse of customer conversations in real-time and resolve issues faster. We have also helped amass 1.5 million voice datasets through community participation to build a voice-enabled interaction system in the Telugu language. Other projects, such as Vaani (a collaboration between the Indian Institute of Sciences and Google), Bhashini (an Indian government initiative to create an open-source Indic language dataset), and 'IndicCorp v2' (an extensive collection of texts developed by AI4Bharat), are also contributing to the development of indigenous LLMs.

Building a Secure, Compliant, and Culturally Aware AI Ecosystem
To conclude, Indigenous LLMs are not just a matter of data security; they represent a strategic approach to managing information in a way that respects local values, adheres to regional laws, and empowers organisations to thrive in a digital landscape. Their development paves the way for more secure, efficient, and culturally aware applications of technology, building trust among stakeholders and leveraging AI to its fullest potential within a legally compliant framework.
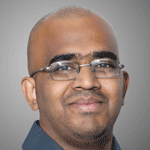
Chaitanya Chokkareddy
Chaitanya Chokkareddy is Chief Innovation Officer at Ozonetel.