
Adapting to change: Synthetic data's rise in Martech's new era
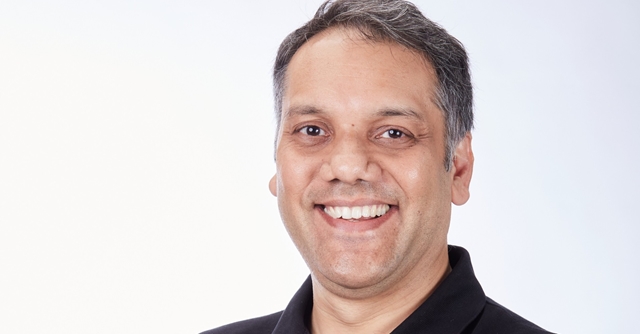

The realm of digital marketing is currently undergoing a seismic shift, with third-party cookies taking a walk into the sunset. Adhering to increased privacy concerns and regulatory changes marketers are scrambling for alternatives. This threatens to create a data void, where the granular insights that once powered targeted marketing campaigns become scarce.
As a result, marketers are now shifting towards first and zero-party data for personalizing customer journeys. However, their impact hasn’t been as substantial.
First-party data is limited to interactions that occur on the brand’s properties, offering a narrow view of customer behavior. Alternatively, zero-party data is dependent on the user’s willingness to share their information. It also has the potential to be rife with biases and inaccuracies, while being difficult to gather at scale as it demands attractive,resource-intensive data collection methods.

Enter, Synthetic Data Generators
At times organizations just don’t have access to enough quality data, either due to the size of the enterprise or the time at hand. What’s the point of this data if it isn’t of a particular quality, or if it lacks critical insights?
Given the limitations of first-party and zero-party data, synthetic data generators (SDGs) emerge as a promising solution. Synthetic data is artificially generated data that mimics the statistical properties of real-world data without revealing any actual user information.

SDGs use various techniques, including machine learning algorithms, to produce data sets that reflect real-world conditions. It helps in replicating, mirroring, or extracting look-alike information that allows analysts to model use cases that would otherwise be impossible to.
For instance, a subscription service company wants to improve customer retention rates. They generate synthetic data that mimics their subscriber base, including sign-up patterns, usage frequency, and cancellation rates. By analyzing this synthetic data, the marketing team can identify key factors influencing retention and design targeted campaigns to enhance customer loyalty.
SDG can also be used for predicting future marketing plans. For example, a fast-food chain planning to launch a new menu item uses synthetic data to create a virtual customer base that reflects the diversity of its real-world patrons. By testing various promotional offers, pricing strategies, and advertising messages in this synthetic test market, they can predict approaches likely to drive the most sales and customer satisfaction when the new item is introduced.

How Synthetic Data Generators Make a Difference
Brands are striving to develop unique, personalized customer journeys – journeys that matter and help maximize customer lifetime value – and, brands need to rely on enough first and zero-party data to power insights that can generate such experiences.
Where zero and first-party data fall short, synthetic data offers a practical solution, enabling brands to generate look-alike data that replicates the characteristics of existing customer data without retaining any identifiable connections to individual customers. Here’s how SDGs improve the overall marketing experience

Privacy Compliance: SDG’s most significant advantage is its inherent privacy protection. Since synthetic data does not contain real user information, it’s compliant with privacy regulations. This makes it an attractive alternative for marketers navigating the increasingly complex domain of data privacy laws.
Data Augmentation: Synthetic data can augment existing data sets, filling gaps where data is missing or sparse. This is particularly valuable for training machine learning models, where large, diverse data sets are crucial for accuracy and reliability, but none are available. Moreover, synthetic data can be produced at scale, enabling marketers to create vast data sets quickly – essential for testing and validating new marketing strategies in a controlled, risk-free environment.
Cost Efficiency: Collecting real data and accurately annotating on a large scale can be an expensive process, while also being time-consuming, especially for team members who support customer journey management processes. Poorly cleaned data can create imbalances, making it harder to train good models for journey-based analytics. Generating synthetic data can be more cost-effective than collecting real-world data, especially when dealing with sensitive information that requires stringent compliance measures. It also reduces the need for extensive data cleaning and preprocessing.

Synthetic Data as a Complementary Solution
While synthetic data presents numerous benefits, it is not a panacea. SDGs should be viewed as a complementary solution rather than an absolute one. They provide a means to enhance and expand upon first-party and zero-party data, rather than replace them entirely.
Combining Data Sources: By integrating synthetic data with first-party and zero-party data, marketers can create a more comprehensive and nuanced view of their audience. This hybrid approach allows for richer insights and more effective targeting strategies.

Mitigating Biases: Synthetic data can help identify and correct biases present in first-party and zero-party data, ensuring that marketing models and strategies are more accurate and representative.
Innovative Testing: SDGs enable marketers to test new strategies and campaigns in a simulated environment, reducing risk and increasing the likelihood of success when applied in the real world.
Cautions along the way
SDGs, despite their abilities, aren’t perfect. Leveraging their full potential, the following cautions must be factored into:
Data Quality and Authenticity: Ensuring the quality and authenticity of synthetic data is paramount. Poorly generated synthetic data can lead to inaccurate insights and flawed decision-making. It's essential to validate synthetic data against real-world benchmarks to ensure its reliability.
Bias in Data Generation: Synthetic data can inadvertently introduce or amplify biases present in the original datasets. If the algorithms generating synthetic data are trained on biased data, they can perpetuate those biases. This can lead to unfair or discriminatory outcomes, particularly in marketing where personalization is key.
Privacy Concerns: One of the significant advantages of synthetic data is its ability to preserve privacy. However, there exists a risk of re-identification. Sophisticated techniques might be able to reverse-engineer synthetic data to reveal sensitive information about individuals, compromising privacy.
Over-reliance on Synthetic Data: Relying too heavily on synthetic data can lead to a detachment from real-world data and insights. Synthetic data should complement, not replace, real data. Marketers must strike a balance to avoid losing touch with actual consumer behavior and trends.
Maintenance and Updates: Synthetic data models require regular updates and maintenance to stay relevant and effective. As real-world conditions change, so must the synthetic data generation processes. Keeping these models up-to-date can be resource-intensive and complex.
Limited Scenarios and Edge Cases: Synthetic data might not always capture rare or edge cases that occur in the real world. These outliers can be critical in certain marketing scenarios. Overlooking them can result in missed opportunities or inadequate responses to unique situations.
Charting the rocky terrain of customer engagement, the true power lies in the synergy between synthetic, first-party, and zero-party data. Together SDG will not only mitigate the challenges of a cookie-less world but also pave the way for a more resilient, innovative, and privacy-conscious future in digital marketing.
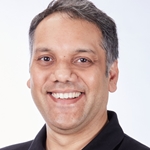
Anand Jain
Anand Jain is Co-Founder and Chief Product Officer of CleverTap.