
How Lendingkart improves efficiency, reduces costs by building a data lakehouse
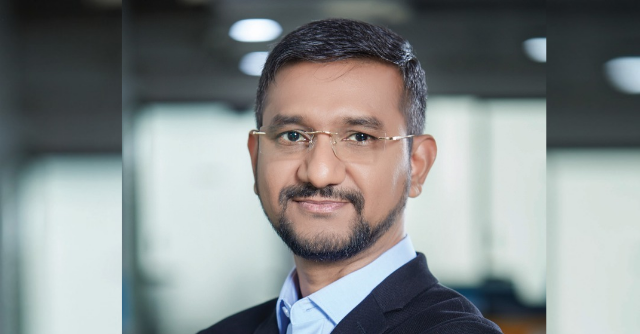

Data forms the cornerstone of any digital transformation journey, with an increasing number of lenders embracing data-driven strategies. Lendingkart, a digital lending platform catering to micro, small, and medium enterprises, has recently implemented a Data Lakehouse architecture that combines the features of data lakes and data warehouses.
Data warehouses are structured repositories tailored for managing and analysing substantial data volumes from various sources, whereas data lakes provide flexible storage for raw and unprocessed data, accommodating structured, semi-structured, and unstructured formats. By leveraging the data lakehouse architecture, companies can combine the adaptability of data lakes with the dependability of data warehousing to establish a cohesive platform for managing diverse data types, therefore enhancing operational efficiency, and customer service, and reducing the overall costs.
The Ahmedabad-based NBFC fintech platform extends loans averaging between ₹5 lakh and ₹6 lakh and has disbursed over ₹18,700 crore to more than 300,000 businesses across 4,100 cities. The cloud-native company already has a strong digital footprint including a loan management system for post-dispersal activities and automated credit underwriting, and it mostly operates online without physical offices considering it serves small businesses in the remotest parts of the country. This also involves a goldmine of data which if catalogued, analysed and interpreted can yield tremendous value.

Giridhar Yasa, the Chief Technology Officer of Lendingkart, explained the challenges that prompted the shift to a data lakehouse. He said, the dispersed nature of data across numerous transactional databases, complicates comprehensive analysis and reporting. Additionally, he said, the absence of Service Level Agreements (SLAs) adversely affected reporting and decision-making capabilities.
Yasa and his team recognised that a data lakehouse could resolve these challenges by consolidating data into a single platform, enhancing their ability to discover and access information.
Speaking about the custom-built technology, he mentioned, “We used Apache Superset for reporting, avoiding commercial solutions that require data transfer outside our network, which poses security and cost risks. To mitigate these, we developed and managed our data pipelines in-house using Java and Scala.” The key infrastructure included AWS MapReduce for our needs and managed Kafka servers from AWS for queuing - a method of requesting specific information from a database using commands, keywords, and expressions.

Discussing the process of implementing the data lakehouse, he said, “Implementing a data lakehouse involved a series of structured steps to ensure effective integration and governance. First, we standardised the format for data storage, which allowed for consistent data organisation and easier access across the platform. We then implemented a data cataloguing solution to manage metadata and improve data discoverability.
Next, the company enriched the metadata with ownership information, clarifying who is responsible for and answering questions about each dataset. To maintain data integrity and security, we established guardrails that control who can publish data and specify the transactional sources from which data can be ingested said Yasa.
The company then developed data pipelines to handle data snapshots, creating two types of SLAs - one for batch reporting with a freshness of one day and another for real-time reporting with a freshness of 15 minutes. This setup ensures that the reporting meets varying needs for timeliness and accuracy. The company also implemented standardised tools for publishing and sharing reports, which facilitate consistent and efficient communication of insights.

“We also rolled out standardised tools for publishing and sharing reports, facilitating consistent and efficient communication of insights. Finally, we built a role-based access control system with custom policies to provide fine-grained governance. This system enhances security, auditability, and overall data management by ensuring that access to data and reports is appropriately restricted and monitored,” he said.
Following the data lakehouse implementation, the company encountered several challenges during and after the process, such as cultural obstacles related to adoption are common.
“To overcome these challenges, we engaged stakeholders through educational sessions and dissemination meetings in a systematic manner,” said Yasa. We also established a regular feedback loop to address issues concerning data publishing and querying, taking into account stakeholder input on the query experience and new feature requests.

That said, to support ongoing use, the IT team developed a stakeholder engagement cadence and enhanced its Help Desk and support portals. This approach ensured that users received the necessary resources and assistance, facilitating a smoother transition and effective utilisation of the data lakehouse.
More importantly, following the implementation of the data lakehouse, the company observed considerable advantages as the time needed to generate new reports was significantly shortened from one week to merely two hours.
At the same time, data volumes surged by a factor of 40, while expenses increased by only 2.5 times, Yasa noted. This improved the efficiency and scalability of our data management strategy.

According to the CTO, the next logical steps for our data lakehouse will focus on expanding its functionalities to facilitate automated workflows for AI/ML training, thereby enhancing our capacity to utilise advanced analytics and machine learning.
“Additionally, we intend to provide self-service analytics for our partners, which include over 20 banks and non-banking financial companies, granting them direct access to insights and reporting, thus further augmenting the value and usability of our data lakehouse,” Yasa summed up.