
Building trust and transparency in the age of automation
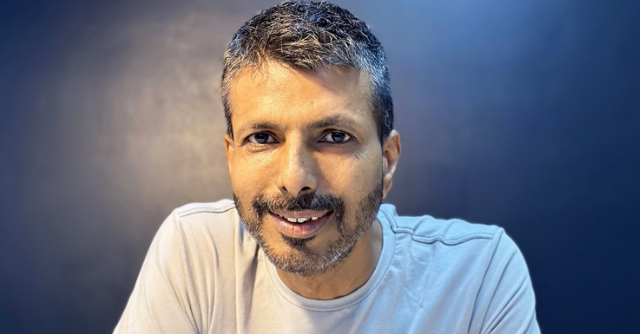

For decades, trust has been the bedrock of successful companies. From the corner store we frequent to the tech giants shaping our digital lives, these brands have built their reputations on consistent quality and a genuine understanding of their customers' needs. However, automation is rapidly transforming how we interact with businesses, from self-service kiosks to AI-powered chatbots. This raises a critical question: can trust survive in an age of automation?
Surveys suggest that consumers are increasingly wary of opaque systems that churn out decisions without explanation, or lengthy ‘terms and conditions’ agreements that are difficult to understand. Forward-thinking companies recognize this and are actively building trust in their automated systems through transparency, inclusivity, and clear communication.
The Opaque AI: Why AI-First Automation Can Erode Trust

The relentless march of automation is transforming every facet of our lives, promising efficiency and progress. Yet, beneath the veneer of convenience lies a potential pitfall—the erosion of trust.
The core of automation, machine learning models are trained on vast datasets and can unintentionally perpetuate societal biases if the data is not meticulously curated. Imagine an algorithm denying loans more frequently to residents of a historically disadvantaged area, regardless of their creditworthiness. This exemplifies the risk of bias infiltrating automation and producing unfair outcomes.
Moreover, many advanced algorithms are intricate "black boxes." Their inner workings are shrouded in complex mathematical operations, making it difficult to understand how they reach a conclusion. This opacity raises questions about accountability. If an automated system makes a mistake, who's to blame for critical errors? Programmers, data scientists, or the algorithm itself?

Building Trustworthy AI-Led Automation: A Multifaceted Approach
Imagine an AI system you can interact with confidently, knowing its decisions are fair, unbiased, and secure. Building ‘trustworthy AI’ requires transparency, user control, and robust safeguards. This ensures AI remains an empowering tool, not a source of apprehension.
The cornerstone of trustworthy AI lies in ensuring fair representation within the training data, while openly discussing the hyperparameters used to train models empowers users by fostering understanding of the system's assumptions and limitations. Transparency extends to pre-trained models as well. Users deserve to be informed about the source data used when techniques like transfer learning or fine-tuning are employed. Furthermore, techniques like regression analysis can provide valuable insights and improve prediction accuracy, ultimately enhancing the user experience.

Building trust in automation goes beyond technical safeguards and necessitates prioritizing data privacy with techniques like anonymization (PII redaction) and encryption to secure sensitive information. For highly sensitive data, private cloud deployment offers increased control over storage and security compared to public cloud options. Ultimately, Users should have full control over their data, with clear options for data collection and precise control over information sharing and usage. Principles like data minimization, which limits data collection to essential needs, and purpose limitation, which clarifies how the data will be used, are crucial for building trust.
Beyond data privacy and control, inclusivity and accessibility are equally important. This means creating user interfaces (UI) that are easy to use for people of all abilities, ages, and backgrounds. For instance, providing alternative text descriptions for images ensures accessibility for visually impaired users. Furthermore, Explainable AI (XAI) empowers users by providing clear insights into how the AI reaches its decisions.
To combat bias, robust validation protocols like 2-way and n-way matches act as ethical checkpoints, verifying the accuracy and fairness of AI solutions and minimizing the risk of biased outcomes. On the security front, constant vigilance is essential to address potential vulnerabilities like prompt injection and toxicity in automation. Employing tools from the Open Web Application Security Project (OWASP) for Vulnerability Assessment and Penetration Testing (VAPT) helps identify weaknesses, while adopting a Secure Software Development Life Cycle (SSDLC) from the outset builds robust defenses against potential security breaches.

By prioritizing transparency, user control, and ethical considerations, we can build AI systems that are not just efficient, but trustworthy partners in progress. By focusing on these multifaceted aspects, we can build trustworthy automation systems that foster trust, transparency, empower users, and contribute to a more inclusive and ethical future.
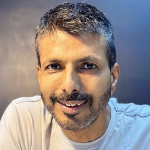
Sanjeev Menon
Sanjeev Menon is Co-Founder and Head of Product & Tech at E42