
We aim at unlocking data and AI value for enterprises: Sujoy Golan, CEO, Multiwoven Technologies
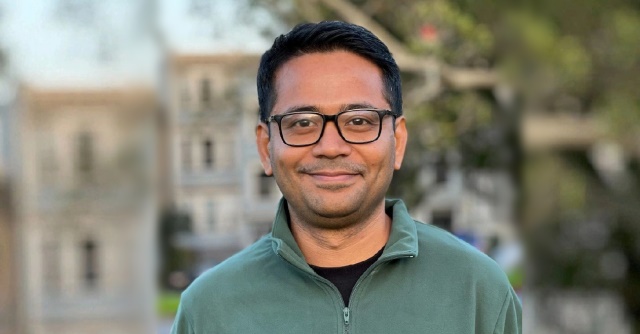

Open-source reverse ETL (Extract, Transform, Load) platform Multiwoven Technologies was acquired by US-based technology firm AI Squared last month, just a year after it was founded by Sujoy Golan, Nagendra Dhanakeerthi and Subin T P. This acquisition comes on the heels of AI Squared's successful $14 million Series A funding round, led by ANSA Capital and NEA. In an exclusive interview with TechCircle, Golan, Co-founder & CEO of Multiwoven Technologies and now Chief Product Officer at AI Squared, discussed the impact of the acquisition on the startup, ongoing projects, and future plans. Edited Excerpts:
What does your company do? Can you briefly explain your offerings?
Multiwoven originated from a team working with large data science teams. We noticed that 80-90% of AI projects in companies never reach the business side, where teams use applications like Salesforce, chat apps, collaboration tools, and marketing platforms. Recognising this gap, we partnered with AI Squared to bridge it by integrating data into business applications.

While AI Squared focuses on data models and insights, Multiwoven emphasises moving data into practical use. We started as an open-source company dedicated to democratizing data movement and quickly grew, finding many synergies with AI Squared. AI Squared helps enterprises maximise the value of their AI and machine learning (ML) investments.
How did AI Squared make this deal happen? What factors made it a compelling decision for your company?
As founders, we blogged extensively, sharing our detailed thoughts. When we started, our goal was to democratise data movement. Data silos are prevalent in data warehouses like Snowflake and Databricks, which are growing rapidly. Business applications are hungry for data, but bridging the gap between data storage and usage is complex.

You spend money creating and gathering data in your warehouse, and your business applications need it. Making this happen is a complex task today. We aimed to simplify this process and make it accessible to everyone. Unlike other companies charging teams to move their data, we believe teams shouldn't pay to move their own data from their own warehouses to their own tools.
We launched our solution on GitHub as open-source and saw immediate traction and adoption from companies. This allowed us to discover new use cases quickly. One significant use case was activating AI, where AI outputs from models needed to be utilised.
We didn't initially focus on AI activation but discovered its importance through customer interactions. The open-source approach facilitated rapid customer adoption, allowing us to uncover pain points quickly. Activating AI insights from existing models was challenging, so we considered two paths: building capabilities ourselves or collaborating with others in the same space.

We met AI Squared CEO Benjamin Harvey (Ben) and his team at an event in Silicon Valley. We realised their vision, especially regarding AI, aligned with ours. We brought the data movement expertise necessary for successful AI activation in business applications.
Before the acquisition, we did a proof of concept (POC) with a large FMCG brand in the US, which went well, and we jointly won the account. Seeing the value we added to customers, we decided to move forward together.
You must already have ongoing projects. How has this acquisition impacted them?

We had two main focuses: actively developing our open-source product and supporting our existing customers.
From the start of our discussions with the AI Squared team, and even after closing the deal, their commitment to these priorities was unwavering. We launched this with a clear message: we will accelerate our open-source development using additional resources, and we will maintain our high level of support and commitment to our current customers.
Both priorities were established early in our discussions with AI Squared and reaffirmed publicly upon closing the deal.

When it comes to AI, in your experience, what are the main reasons enterprises struggle to get value from their investments?
First, many companies have recently invested heavily in generative AI. Some have purchased software to automate tasks like customer support and marketing, while others have built internal teams to develop AI solutions. However, these rapid investments are often not well-aligned with business objectives, driven instead by a desire to keep up with trends.
Second, companies with sustained AI investments over the past five or six years have focused on building large data science teams. These teams create valuable models for credit scoring, product recommendations, and lead scoring. Despite these efforts, the business teams still rely on existing applications, leading to a disconnect between AI outputs and business operations.

Finally, these disconnects between AI and business applications result in inefficiencies. For example, sales teams continue using platforms like Salesforce, while marketing teams use tools like Moengage or Brace. Similarly, financial services stick to their loan management systems. Bridging these two worlds is crucial for realising the full value of AI investments.