
We are investing heavily in Gen AI for innovation across industries: Anurag Sahay of Nagarro
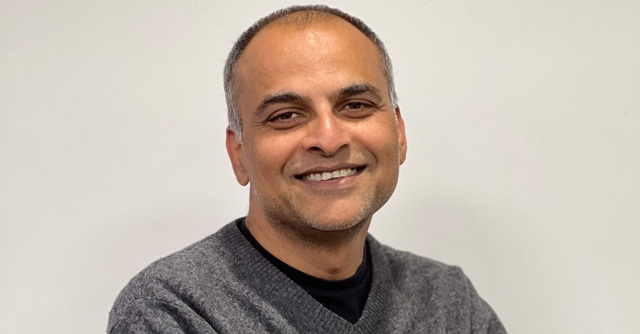

Digital product engineering company Nagarro has been facing headwinds like many global tech peers due to the discretionary project-based nature of digital business. In the third quarter ended September 30, 2023, it managed just over 6% year-on-year constant currency growth in global business. But the firm that is just short of a $1 billion annual revenue-mark, sees the excitement around artificial intelligence (AI) tempting clients into conceptualising data and AI projects that they would not have considered a year ago and it continues to attract new clients via these hot domains. It expects that the improved capabilities of AI solutions will drive a race in each industry to harness this new technology to improve customer experience and delivered value, to win growth and market share, and to enhance safety, security and efficiency. Naturally, a good quantum of digital engineering work will be required to enable these activities. TechCircle spoke to Anurag Sahay, Nagarro's Managing Director of AI and Data Science, to gauge emerging trends, challenges, impact of AI on business applications and more. Edited Excerpts:
What are the emerging technological trends that you see or anticipate will shape the future of IT industry in the coming years?
Certainly! In the evolving landscape of AI, a few key trends are reshaping the industry. Firstly, there's a notable rise in intelligent platforms, with SaaS (software-as-a-service) services leading the charge. Traditional enterprise systems are transitioning to cloud-based solutions, enhancing their intelligence. Notably, Salesforce and ERPs are now more intelligent, reflecting a broader shift in enterprise applications.
Secondly, the emergence of data platforms is transforming how we handle information. Platforms like Snowflake and Databricks exemplify a new breed, fostering ecosystems and developer communities. The concept of data as a platform is gaining traction, akin to the success of Android or iPhone in the mobile space. Lastly, a significant trend revolves around enterprises leveraging generative AI to create value. The integration of large language models like ChatGPT with proprietary data is becoming a strategic move. This synergy aims to tailor solutions to specific enterprise needs, a shift beyond the initial use of open-source models like ChatGPT, Anthropic, and Gemini.
In summary, the industry is witnessing a shift towards more intelligent platforms, the rise of data-centric ecosystems, and a strategic focus on combining generative AI with enterprise data to deliver tailored value propositions.
Have you noticed any specific advancements or trends in AI and data science, given the evolution you mentioned? Are these becoming increasingly vital for enterprises, and how is your company adapting to them?
In the realm of data science and AI, particularly within enterprises, three key applications stand out. First, there's business intelligence, revolutionising how businesses manage operations through insightful reporting and analytics. Second, real-time streaming applications provide almost instant responsiveness, exemplified by app recommendations based on immediate user actions. Lastly, data science employs mathematical and computational techniques to achieve specific objectives, while AI, especially in generative AI, represents a significant emerging area. Across business intelligence, real-time streaming, data science, and AI, remarkable evolution is evident. Business intelligence incorporates generative AI and root cause analysis, real-time streaming approaches near-instant responsiveness, and data science sees radical changes in forecasting, optimisation, and predictive modelling. Advances in performant architectures and scalable cloud solutions make these applications easier to build. The landscape of AI, particularly generative AI, marks a notable evolution in these dynamic domains.
But do you see any unique challenges in the enterprise setting when you're applying generative AI technology?
There are several challenges to consider here. Firstly, achieving a consolidated data infrastructure is the key. Many companies have scattered data across various platforms like data lakes and warehouses, making it difficult to harness the power of AI effectively. Without a unified approach, leveraging technologies like generative AI becomes significantly more challenging.
Secondly, integrating this data requires a robust governance and security framework. Access must be carefully managed to ensure the right people can utilise the data without hindrance. Balancing accessibility with security is crucial for enabling value creation.
Thirdly, there's often a lack of awareness and understanding around AI technologies. Many enterprises lack a clear roadmap for implementation, leading to fragmented efforts and abandoned projects. Without a coherent strategy, realising the full potential of AI remains elusive for many organisations.
Addressing these challenges is fundamental for enterprises looking to harness the transformative potential of AI effectively.
When it comes to data and training generative AI models, there's a common discussion, especially in India, about data scarcity during the model-making process. What's your take on this?
Addressing data scarcity is a common challenge for organisations considering model fine-tuning or building from scratch. Overcoming this hurdle is feasible with a clear intention and recognition of the value in developing datasets. Historically, AI models were often built using Supervised Learning, where input-output pairs formed datasets for training. This approach required meticulous compilation of datasets for specific use cases. Generative AI and large language models, such as GPT-3, have altered this landscape. These models are massive, requiring trillions of tokens for training, equivalent to almost a million Wikipedias. Access to such extensive data is a limitation for many companies.
Even when not training an entire model from scratch, fine-tuning large language models demands high-quality data. Quantity alone is insufficient; the emphasis is on quality. Creating a dataset for effective fine-tuning involves careful consideration of desired attributes, adding complexity to the process.
However, in applications like retrieval augmented generation, organisations can leverage large language models without fine-tuning. By combining these models with small enterprise data using a prompt, organisations bypass the data scarcity issue. This approach, known as in-context learning, is gaining popularity as it eliminates the need for extensive fine-tuning or building from scratch.
Looking ahead, organisations invested in fine-tuning will likely dedicate efforts to build high-quality datasets, even if it means eventually training large language models from scratch.
What are the specific challenges or limitations in acquiring real-world data in certain situations, and how do you overcome them?
Considering the current landscape, there are already thousands of large language models available on platforms like Hugging Face. Companies like MosaicML offer the creation of such models for around 50K to 100K. Obtaining real-world data might require effort, but it's readily available.
Looking ahead, challenges may arise as companies invest and competition in the AI space intensifies. However, an intriguing possibility involves integrating robotics with language models. Imagine robots collecting diverse data in the real world, contributing to datasets for various applications. While this presents challenges in terms of energy and cost, our creative capacity as a society will likely drive us to overcome these obstacles. In essence, the evolution of AI is unlikely to halt or slow down due to data limitations.
How does your company stay ahead in adopting the latest generative AI advancements for enterprise users, given the evolving nature of artificial intelligence?
Certainly! At our company, expertise in AI is cultivated at different levels. Firstly, our dedicated engineers are passionate and self-driven, investing personal time to stay updated on developments. Over the past 8 to 10 years, we've strategically invested in AI, forming a solid foundation.
Next, our partner ecosystems play a vital role. Collaborating with companies globally, particularly in Europe, the US, and India, allows us to stay current on Gen AI, data consolidation, integration, and AI trends.
Lastly, our learning-by-doing approach involves active participation in diverse projects—ranging from Gen AI to machine vision and data science. Accumulating experiences from these projects forms the bedrock of our knowledge and insights. Together, these levels have propelled us to our current position.
Could you tell us more about your current research or future plans at the company, especially regarding Gen AI or any other applications?
We're heavily investing in generative AI, with three dedicated practices: prompt engineering, retrieval augmented generation, and fine-tuning large language models. Engaging with open source models like Lama, Mistral, Alfalfa, we anticipate expanding our generative AI efforts. We're strategically merging generative AI with our expertise in banking, aviation, automotive, retail, and more. Our 'fluidic enterprise' program is aimed at enhancing domain expertise and technological readiness across verticals. Additionally, we've developed accelerators and partial products to better understand market needs and optimise Large Language Models for value and ROI. These efforts will unfold in the coming months and years at Nagarro.
Is your company exploring the Metaverse too? This technology has quickly gained popularity, similar to generative AI. However, there seems to be limited activity, particularly in India. How do you foresee the Metaverse influencing the IT industry in the near future?
Recently, Apple launched the Apple Vision Pro, an impressive piece of technology gaining attention. Videos show people using it for immersive experiences, and major players like Apple and Meta are investing heavily in this direction. The Metaverse seems destined to become more pervasive in the coming years. While predicting its widespread adoption is uncertain, our IT industry engagement includes various proof of concepts and prototypes. We anticipate continuing such initiatives as they gain enterprise attention. Though the exact timeline is unclear, we remain committed to investing in and optimising these technologies, eagerly awaiting market developments in this direction.