
Cloud native tech is a crucial component of our innovation: Lentra's CDO, Rangarajan Vasudevan
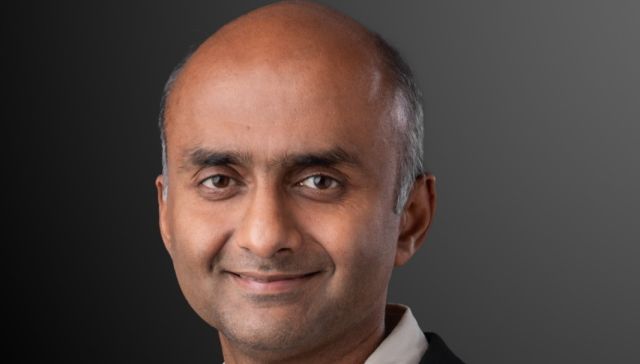

Lentra, a digital lending platform, recently introduced its Intelligent Lending Cloud Platform, marking an advancement in incorporating artificial intelligence (AI) into digital lending. In an interview with TechCircle, Rangarajan Vasudevan, Chief Data Officer at Lentra, shared insights about the newly launched features and the practical implications for fintech, banks, and other financial institutions.
Edited Excerpts:
How do the new features, like Convo, Insights, and Wingman, benefit lenders?

Convo enhances financial inclusion by facilitating conversations in various languages, enabling wider access to loan products. It addresses the challenge of making loan products accessible to diverse populations with different linguistic preferences and cultural nuances.
Moving on to the second product, Insights, designed for back-office operators. It simplifies the decision-making process by optimizing parameters for lending, helping lenders expand their business opportunities while managing risks effectively. This is particularly useful for lenders with specific themes or theses, such as focusing solely on farmers.
Lastly, there's Wingman, a product tailored to streamline the work of underwriters—the key decision-makers in the lending journey. It automates the processing of vast amounts of data, providing clear recommendations for straightforward cases and raising intelligent questions for those in the grey area. This significantly saves time for underwriters, allowing them to focus on applications that require closer examination.

How does fraud detection play a role in your products and services, particularly concerning loans?
Fraud detection is an integral part of our base platform, essential for any lending journey through us. It extends beyond the three featured products, covering various types of fraud. Identity fraud involves someone posing as another person, either through stolen identity or fake ID proof. Income fraud occurs when an individual claims a higher income than reality to secure a loan. Contact ability fraud involves providing false contact information, affecting loan recovery efforts. Additionally, there's asset-specific fraud, tied to the type of loan product. For instance, collusion with a retailer to obtain a loan for a product not eligible for financing.
Our lending platform employs verification processes and collaborates with government data sources, like passport and Aadhar data, to address these fraud types. However, innovation is ongoing. For example, we've implemented a feature over the past two years to combat asset-specific fraud. We record the unique device number (IMEI) of phones financed through us, informing manufacturers like Samsung, Apple, etc. This real-time tracking helps identify and prevent fraudulent activities, showcasing our commitment to constant improvement and customer protection.

Can your wingman feature effectively handle situations where users may attempt to cheat by using products like ChatGPT for providing plain answers to tricky questions, thus compromising the evaluation process?
The wingman feature adeptly manages both clear-cut scenarios and those with uncertainties. In the grey area, it refrains from making decisions independently. Instead, it presents five reasons for its indecision, prompting the underwriter to intervene. The underwriter evaluates the consumer's responses, as the tool merely facilitates the process without making direct lending decisions. For instance, the wingman highlights the issue in cases where discrepancies arise, like a claimed Rs 1 crore turnover versus a calculated Rs 60 lakh based on financials. However, it does not outright reject or approve, leaving it to the underwriter's judgment to delve into potential explanations, such as seasonal variations or unforeseen circumstances. The wingman serves as a guide, focusing the underwriter's attention on critical aspects for a final lending decision by the bank.
How accurate are these features?

We prioritize caution in our approach. Our clients operate under regulations, requiring justification for their decisions, even at the level of individual loan applications. We lean towards caution, allowing lenders to determine the acceptable risk level associated with automation. The definition of a grey area is flexible, with configurable lower and upper thresholds. Lenders can adjust the range based on their risk preferences, allowing them to make informed decisions.
Can you share insights into how these AI features were developed? In the context of India's data scenario, where there's a discussion about data availability for training AI features, how did you tackle this while building these three features?
There is a scarcity of data in specific segments, but it's not a hindrance for other segments. In the current AI wave, centered around large language models (LLM), the model learns probabilities. It understands the likelihood of a word appearing next in a sequence, considering language nuances. When practitioners mention a lack of data, they refer to insufficient digitized text for languages like Sanskrit or Tamil. However, in our domain, dealing with transactional data stored in databases as bits and bytes, there's an abundance. For instance, every cash, ATM, or credit card transaction, processed in English, provides a rich dataset for credit modeling.
