
MIT, Cohere for AI, others launch platform to enhance transparency in AI data
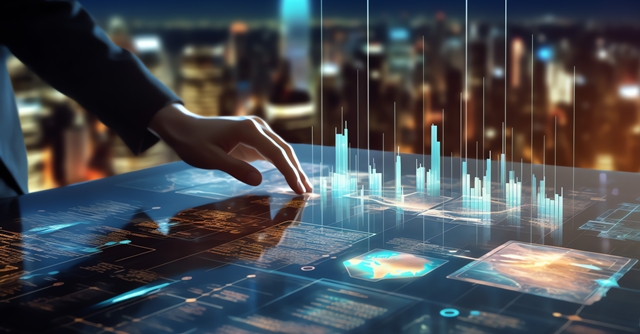

Researchers from the Massachusetts Institute of Technology (MIT), research lab Cohere for AI and 11 other institutions unveiled the Data Provenance Platform on Thursday aimed at addressing the data transparency crisis within the artificial intelligence (AI) field.
The researchers embarked on a thorough examination and tracking of almost 2,000 of the most widely utilised fine-tuning datasets. These datasets, collectively downloaded millions of times, form the foundation of numerous significant NLP advancements, as outlined in a message from Shayne Longpre, a Ph.D. candidate at MIT Media Lab, and Sara Hooker, the head of Cohere for AI.
The result of this collaborative endeavor stands as the most extensive audit of AI datasets to date. Significantly, these datasets now feature tags that lead back to their original data sources, details about re-licensing, the creators, and other data attributes.

To make this invaluable data more accessible, the team has introduced an interactive platform, the Data Provenance Explorer. This tool allows developers to track and filter through thousands of datasets to ensure compliance with legal and ethical standards. Moreover, it empowers scholars and journalists to delve into the composition and lineage of popular AI datasets.
In conjunction with the platform launch, the group released a comprehensive paper titled "The Data Provenance Initiative: A Large Scale Audit of Dataset Licensing & Attribution in AI." The paper emphasizes the prevailing issue where commonly used dataset collections are treated as unified entities instead of a lineage of data sources, collected, curated, and annotated with successive rounds of repackaging and re-licensing.
Failure to acknowledge this lineage is often due to the magnitude of modern data collection and heightened copyright scrutiny. This neglect has resulted in fewer datasheets, a lack of disclosure of training sources, and ultimately, a diminished understanding of training data.

This lack of comprehension can result in data leaks between training and test data, the exposure of personally identifiable information, unintentional biases or behaviors, and models of lower quality than initially anticipated. Beyond these practical challenges, the absence of information and documentation carries significant ethical and legal risks, such as model releases conflicting with data terms of use. Given the expense and irreversibility of training models on data, addressing these risks and challenges is no straightforward task.