
Tech Mahindra aims to create foundational language model rooted in India: Nikhil Malhotra
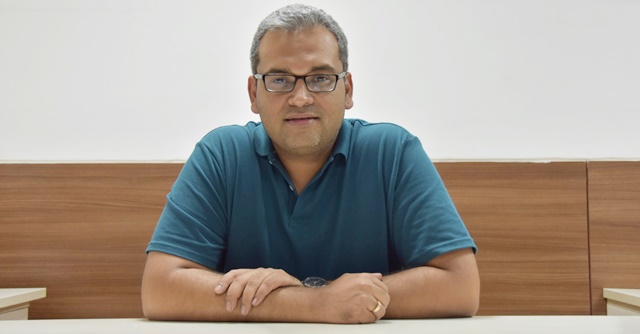

In June, Tech Mahindra's CEO CP Gurnani and OpenAI's CEO Sam Altman engaged in Twitter exchanges, signalling a new competition in ChatGPT with an Indian version. Tech Mahindra went on to unveil Project Indus a few weeks later, aiming to create a foundational language model focused on Indian languages, especially Hindi and dialects. It follows an open-source approach, emphasising diverse data sources, seeking to offer accurate, relevant and responsible AI across various domains. TechCircle spoke to Nikhil Malhotra, Global Head-Makers Lab at Tech Mahindra, to dive deeper into Project Indus with its approach to addressing generative AI challenges.
Edited Excerpts:
What sets the project stand out from other AI models?
Project Indus, as the name suggests, is a civilisation initiative for India. We have two primary objectives — first, we aim to create a foundational language model rooted in India. We are currently determining the specific parameters, but we're leaning towards a range of 14 to 40 million parameters for this study. Second, we aim to excel in various benchmarks prevalent in the market, ensuring optimal model performance.
The motivation behind this initiative stems from the fact that while there are numerous language models available today, they predominantly cater to languages like English, German, French and Spanish. In contrast, Indian languages are notably underrepresented. In India, only 10 to 20% of the population speaks English, highlighting the linguistic diversity of our nation. India boasts around 20 to 23 major mother tongues and 1,645 dialects that are actively used. In total, there are approximately 19,500 dialects in India.

While efforts have been made in the field of Indic NLP, there has been no foundational model for India or Indian languages. Project Indus aims to fill this void. Our primary objective is to develop a robust language model based on Indian languages. The choice of which language to start with was a complex decision. However, we decided to commence with Hindi due to its extensive user base, with approximately 615 million speakers across the country, surpassing the number of English speakers worldwide.
Furthermore, we intend to include various dialects of Hindi in our model. Hindi itself encompasses around 38 to 40 dialects, including Dongri, Kinnauri, Kangri, Garhwali, Kumaoni, Braj Bhasha, Bundeli and Awadhi, among others. The reason for incorporating these dialects is to ensure that our foundational model represents the rich linguistic diversity of India comprehensively. Failing to include these dialects would result in underrepresentation and hinder our ability to create models that cater to the needs of the Indian population and our customers interested in Indian languages.
Project Indus is an open-source initiative, and our primary focus is on building the first Hindi-based foundational model, enriched with approximately 36 to 40 dialects. Once accomplished, we plan to expand our efforts to encompass other languages as well.

How do you plan to collect and prepare the extensive amount of data needed to train a language model for India, given the country's linguistic diversity?
We have two data strategies in play here. Firstly, we acquire data from various online sources, including critical ones like Common Crawl, which provides website data. However, the challenge lies in finding dialect-specific data, as most sites primarily offer data in mainstream languages.
To address this, we've established projectindus.in, a portal where people can contribute data in their own languages through “Bhasha Daan”. Here, we gather data and text in various dialects. But our data strategy involves more than just collection; it comprises two key steps.

Step one is data collection, while step two is diversification. Research has shown that models trained on diverse datasets outperform those trained on a single type of data. Our diverse dataset includes content from sources like newspapers, Wikis, and specific domains like construction, general awareness, and news.
Since much of this data isn't readily available in Hindi and English, our initial approach is to collect data in English, translate it into Hindi, and assess it for annotation quality and ethical bias. This annotation process involves human input for refining the data.
Once we've refined this content, which may amount to, for instance, 100-200 million records (hypothetically), it serves as input for our model. Now, let's delve into our model, which involves foundation models and techniques such as reinforcement learning through human feedback.
When discussing Generative AI and its potential for misinformation and fake news, how does Tech Mahindra plan to address these concerns while developing Project Indus?

When it comes to generative AI, there's a concern about potential inaccuracies in responses, which is often seen in models like Chat GPT. At Tech Mahindra, we take two approaches to address this issue, involving both R&D and customer interactions.
First, when dealing with customers, we contextualize the data and establish guidelines to ensure accurate and appropriate responses. We also employ fact-checking measures to verify the information provided. For example, in our Project Indus, which relies on external data, we are careful not to incorporate unreliable information that could lead to inaccuracies or hallucinations.
Let's illustrate this with an example: In Project Indus, we avoid creating a model in Hindi that generates code with potential copyright issues, such as copying existing code. Instead, we focus on gathering information available in the public domain, typically from websites and other verified sources. We also add a second layer of human annotation to validate the data's relevance and accuracy.

When users interact with our model, there's a feedback loop involving reinforcement learning. Users ask questions and provide feedback to help improve the model's responses and prevent hallucinations. Our goal is not to provide unchecked information but to serve a specific segment, like rural finance, while ensuring accuracy and relevance in the responses.
In essence, we aim to strike a balance between leveraging generative AI for practical applications and maintaining safeguards to prevent misinformation and inaccuracies. It's a challenging task, but it aligns with our commitment to responsible AI usage.
So, this will be somewhat distinct from the OpenAI Chat GPT we're familiar with?
Absolutely, it won't function like an all-purpose OpenAI chatbot. Instead, we're focusing on specific domains. Our goal is to ensure that these domains are not only covered but also accurately represented, without generating false information.
What lessons have you learned from ChatGPT's successes and shortcomings that you will incorporate into your project?

In terms of technology, we were thrilled when we first encountered ChatGPT. After two decades of research in natural language processing, the dream of generating language was finally becoming a reality. ChatGPT marked a significant milestone by demonstrating how generative AI could achieve this, and it's been a game-changer for the industry.
However, there are some challenges to address. One major concern is the potential for the model to produce incorrect or hallucinated information. We aim to enhance the model's contextual and factual accuracy, particularly in generating responses.
Additionally, ethical considerations are crucial. We want to ensure that the model does not generate harmful or unrelated content. This includes preventing questions related to dangerous activities or inappropriate topics right from the start.
In summary, ChatGPT represents a significant advancement in generative AI technology. While we appreciate its strengths and the opportunities it offers, we are actively working on implementing guardrails to enhance its accuracy, ethical behavior, and safety.
How will Project Indus be made available to users? Will there be any limitations on who can use it?
This is an open-source project, meaning it will be accessible to everyone. We'll allow anyone to explore and interact with the model from an open-source standpoint. Additionally, users will have the option to download and use the model on their own premises, subject to specific criteria such as having sufficient computing power. In essence, it's an open-source initiative without limitations.
How will Project Indus contribute to the growth and advancement of generative AI in India?
The project has several key goals. First, we aim to include lesser-known Indian languages that are often overlooked. Second, India lacks a foundational model, and we want to fill that gap. Third, we're excited about the potential use cases, such as helping farmers access information in their language, aiding children in their education, and enabling coding in various languages. Additionally, our project, BHAML or Bharat Markup Language, empowers kids to code in their preferred language. Fourth, language barriers often hinder innovation and ideas, so we want to provide India with the language support it needs. Lastly, our model can benefit various sectors like rural finance, retail, and logistics, fostering growth and development across India.