
Genpact's Sreekanth Menon on AI adoption, GenAI use cases, and more
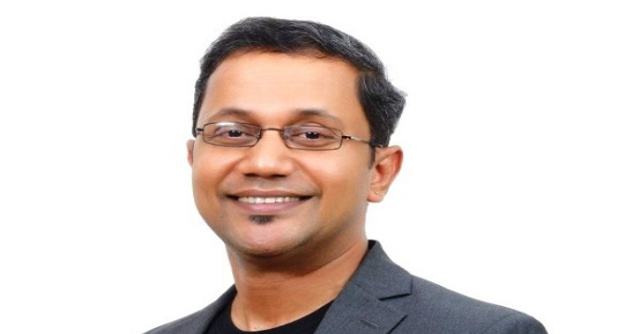

As generative AI (GenAI) continues to grow quickly in various industries, IT company Genpact is dedicated to assisting its clients with their AI strategies. They're also using AI, including gen AI and other principles, to improve processes. In an interview with TechCircle, Sreekanth Menon, Genpact's Global AI/ML Practice Head, discussed the company's AI strategy and plans for GenAI, as well as the significance of responsible AI. Edited excerpts:
How are LLMs or other AI advancements shaping out services at Genpact?
We've had an AI-enabled infusion center of excellence (CoE) for close to a decade now. We started an entire big data boom, and then machine learning came. We have done focused investments in AI/ML and acquired a company called Rage Frameworks in the NLP space (Natural language Processing), way back in 2018. From there, we recognized the potential in small language models and a technique known as the multilayer perceptron (MLP) neural network. This acquisition helped us create what we now call GenAI. So, for us, it is not new, but now with the advancements which we saw in GPT 3.5 and 4, the entire world woke up to the large language model. We are also using a large language model to understand and see how we can infuse in the larger operations. We now have a strategy for certain business verticals and certain business functions. So, at the intersection of industry and domain, we apply AI, and we are reimagining the process with the AI-led approach, which means we are bringing GenAI and other AI tenets to make this entire thing happen. As a strategy, we are looking at customer care, customer services, sales, and commercial, financial and accounting, and tech services.

What kind of investment have you made in AI-related technologies and what gains have you noticed?
It's too early to say but with almost 80% of our existing customers joining us on a journey where we start with small steps but dream big. This means we're trying out new ideas to see how they work and what impact they have. We're taking things step by step, first testing our ideas with AI, then showing our clients how to gain their confidence before fully committing. Right now, we're in the process of testing our ideas and soon we'll be putting them into action. As we do this, we're realizing that our GenAI technology is helping our clients in more ways than one. For example, a global pharma giant had a marketing analytics strategy. We developed a tool to analyze various market signals, like social media, to enhance their productivity. This tool quickly processes data, making tasks faster. Additionally, we improved order fulfillment processes. For instance, the order management team can now provide real-time updates by efficiently handling unstructured data. Another project involved streamlining a client's supply chain contract. Instead of manually searching through pages, our system responds to queries, transforming the way contract management works.
The focus should be on cultivating a responsible AI culture. With GenAI, ensuring responsible decisions from the algorithm is crucial. This requires ethical guidelines and auditing to prevent data leaks and infringements. We assist clients in implementing this. Additionally, we establish a GenAI Center of Excellence for clients, aiding them in kickstarting their enterprise journey. Genpact plays a pivotal role in creating these centers of excellence.

How does Genpact ensure ethical and responsible use of AI?
Responsible AI has many layers, one is related to the industry and brand, and another concerns the client's perspective within the community and globally. Our framework allows us to select and address these responsible AI angles effectively. For instance, domain-specific metrics are crucial for a clear responsible framework, including managing data and model changes, ensuring model reliability and safety, as well as evaluating privacy and security risks. Additionally, explainability and traceability are vital; models must be transparent, enabling explanations for regulatory and compliance purposes. In short, our framework covers industry-specific and client-centric considerations, focusing on metrics, reliability, privacy, and explainability.
Lastly, let's talk about fairness and legal compliance. Our framework starts by helping clients define their vision statement. What they want to achieve is our focus. We then turn that vision into a strategy, bring it to life, and operationalize it. This requires setting up a Responsible AI CoE, establishing guardrails, and considering different personas. And so, we are looking at a very customized responsible AI, because it cannot be out of the box, and it is customized and tailored to every customer.

What are your future plans for technologies like generative AI and ML? Especially in terms of new services and solutions for enterprises?
We are obviously on a growth track for our data and AI business, and we plan to double down our business in the next three years, of analytics, data, and AI business. With that, generative AI and the entire AI ecosystem will play a key role.
We're going to invest a few million dollars in this. And that's why there will be focused partnerships with tech giants because we can't do it without them. Two, focused clients and focused offerings on end-to-end data and AI together. While the point solution will be there, now gen AI has given a boost to think entire end-to-end AI.

We understand talent is very critical for this to happen. We understand, at any point in time, in the near future, everybody will be an AI user. They are going to consume an output of an AI/Ml system at some point in time in a corporate setup like ours. However, this also means ongoing training is needed to ensure everyone understands AI. Currently, we're training over 25,000 people (out of our total 115K) in generative AI using our Genome learning platform. This collaborative platform enables scalable reskilling, magnifying transformation through collective intelligence. Additionally, we're focusing on enhancing domain knowledge, a vital aspect of any AI project.