
Five generative AI strategies for CIOs
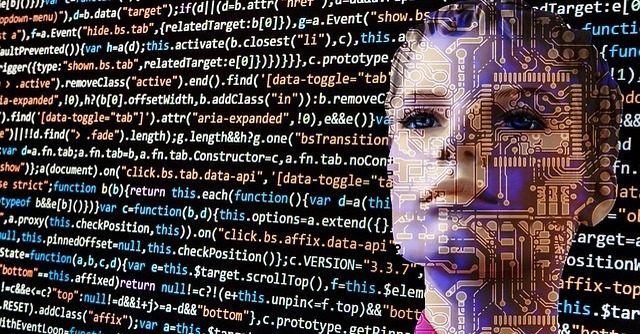

As Chief Information Officers (CIOs) and chief technology officers (CTOs) navigate the ever-evolving technology landscape, generative artificial intelligence (AI), is one technology that demands attention. The AI system capable of generating text, images, or other media in response to prompts, has gotten widespread attention, especially since OpenAI-backed ChatGPT was launched in November last year and many big tech firms launched several plans around the technology soon after.
A report published by consulting firm McKinsey estimated that generative AI could add the equivalent of $2.6 trillion to $4.4 trillion of value annually for enterprises. In a paper published on July 11, McKinsey researchers listed out some ways in which CIOs can harness the power of generative AI to achieve competitive advantage, enhance operational efficiency, and drive digital transformation.
Identifying the right use cases

CIO/CTOs should identify the most valuable opportunities and issues across the company that can benefit from generative AI and those that are not. The use cases may vary from one area to the other. McKinsey researchers, for example, said that generative AI can lift productivity for certain marketing use cases - for example, by analysing unstructured and abstract data for customer preference, by roughly 10% and for customer support roles, through intelligent bots, by up to 40%.
CIOs should develop a perspective on how best to cluster use cases either by domain (such as customer journey or business process) or use case type (such as creative content creation or virtual agents) so that generative AI will have the most value.
“Providing this level of counsel requires tech leaders to work with the business to develop a FinAI capability to estimate the true costs and returns on generative AI initiatives,” said researchers.

Reviewing gen AI’s potential impact on all areas of tech
McKinsey research shows generative AI coding support can help software engineers develop code 35% to 45%, and perform code documentation up to 50% faster. It can also automate the testing process, allowing teams to develop more-resilient software prior to release, and accelerate the onboarding of new developers, say, by asking generative AI questions about a code base.
Likewise, technical debt can account for 20% to 40% of technology budgets and significantly slow the pace of development. CIOs and CTOs should review their tech-debt balance sheets to determine how generative AI capabilities such as code refactoring, code translation, and automated test-case generation can accelerate the reduction of technical debt.

A focus on quickly building generative AI capabilities in software development, accelerating technical debt reduction, and dramatically reducing manual effort in IT operations, said researchers.
Upgrading the tech architecture
Organisations can use many generative AI models of varying size, complexity, and capability. To generate value, however, these models need to be able to work both together and with the business’s existing systems or applications. For this reason, building a separate tech stack for generative AI creates more complexities than it solves, said researchers.

They also suggest companies should upgrade their enterprise technology architecture to integrate and manage generative AI models and orchestrate how they operate with each other and existing AI and machine learning (ML) models, applications, and data sources.
For that CIO/CTOs can develop a data architecture to enable access to quality data by processing both structured and unstructured data sources. For this, creating a centralised, cross-functional generative AI platform team to provide approved models to product and application can help in this regard, they noted.
Investing in upskilling programs by roles and proficiency levels

Generative AI has the potential to massively lift employees’ productivity and augment their capabilities. The benefits, however, may vary, said researchers. Highly skilled developers saw gains of up to 50% to 80%, while junior developers experienced a 7% to 10% decline in speed.
“That’s because the output of the generative AI tools requires engineers to critique, validate, and improve the code, which inexperienced software engineers struggle to do,” they said.
CIO/CTO therefore needs to work alongside the HR head to rethink their talent management strategy to build the workforce of the future. There should be a focus on upskilling people based on a clear view of what skills are needed by role, proficiency level, and business goals.

Beyond training tech talent, the CIO and CTO can play an important role in building generative AI skills among non-tech talent as well. The research shows, in less technical roles, such as customer service, generative AI helps low-skill workers significantly, with productivity increasing by 14% and staff turnover dropping as well, according to a previous study.
Besides understanding how to use generative AI tools for such basic tasks as email generation and task management, people across the business will need to become comfortable using an array of capabilities to improve performance and outputs.
Evaluate the new risk landscape
Researchers noted that generative AI presents a fresh set of ethical questions and risks, including “hallucinations,” whereby the generative AI model presents an incorrect response based on the highest-probability response; the accidental release of confidential personally identifiable information; inherent bias in the large data sets the models use; and high degrees of uncertainty related to intellectual property (IP).
According to McKinsey researchers, CIOs and CTOs will need to become fluent in ethics, humanitarian, and compliance issues.
Addressing this new landscape requires a significant review of cyber practices and updating the software development process to evaluate risk and identify mitigation actions before model development begins, which will both reduce issues and ensure the process doesn’t slow down, they said.
Further, to protect data privacy, it will be critical to establish and enforce sensitive data tagging protocols, set up data access controls in different domains (such as marketing, HR compensation data), add extra protection when data is used externally, and include privacy safeguards. For example, to mitigate access control risk, some organizations have set up a policy-management layer that restricts access by role once a prompt is given to the model.
According to researchers, early generative AI use cases should focus on areas where the cost of error is low, to allow the organisation to work through inevitable setbacks and incorporate learnings.