
IIT Mandi develops new ML algorithm for accurate prediction of landslides
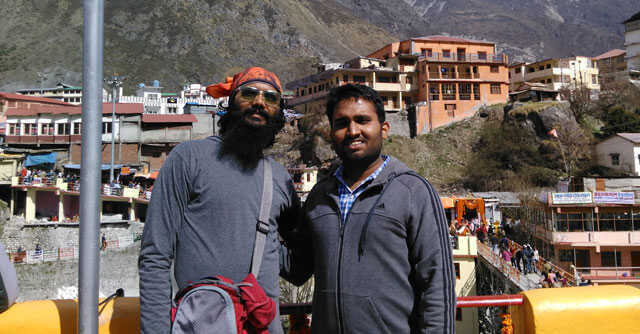

Indian Institute of Technology, Mandi has developed a new machine learning algorithm that improves the accuracy of natural hazard prediction. The proposed solution deals with the challenge of data imbalance in determining the likelihood of landslide occurrence in a given area.
The solution was developed by Dericks Praise Shukla, Associate Professor, School of Civil and Environmental Engineering, IIT Mandi along with Sharad Kumar Gupta, former research scholar, IIT Mandi, currently working at Tel Aviv University (Israel).
The first step in estimating and mitigating the risk of landslides is to identify the most susceptible areas. It is done through Landslide Susceptibility Mapping (LSM) which denotes the likelihood of a landslide occurring in a specific area based on factors such as slope, elevation, geology, soil type, distance from faults, rivers, and faults, and historical landslide data. The use of machine learning techniques makes helps in the accurate prediction of landslide occurrences. However, since landslides are a rare occurrence, it leads to the unavailability of adequate training data.

The new machine learning algorithm developed by Shukla’s team deals with the issue of this data imbalance using two undersampling techniques – EasyEnsemble and BalanceCascade. For developing this algorithm, the team collected data on the landslides that occurred in the Mandakini River Basin in northwest Himalaya, Uttarakhand, India, between 2004 and 2017 to train and validate the model.
“This new ML algorithm highlights the importance of data balancing in ML models and demonstrates the potential for new technologies to drive significant advancements in the field. It also underscores the critical need for large amounts of data to accurately train ML models, particularly in the case of geohazards and natural disasters where the stakes are high and human safety is at risk,” said Shukla.
The proposed solution improves the accuracy of LSM compared to other techniques such as Support Vector Machine and Artificial Neural Network. As per the team, this method can be applied to other natural hazard predictions like floods, avalanches, and extreme weather events.
