
Qualcomm launches 5G chipsets for budget and mid-segment phones
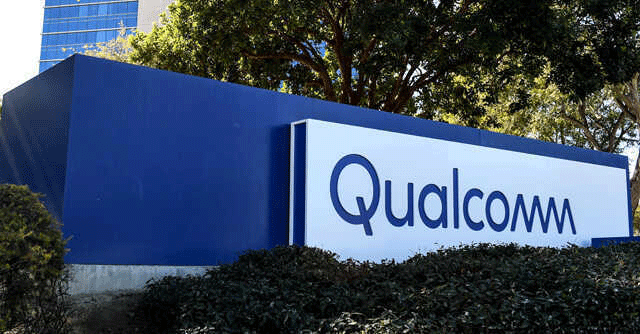

Qualcomm Technologies has launched two new mobile chipsets, Snapdragon 6 Gen 1 and Snapdragon 4 Gen 1, bringing 5G capabilities to smartphones in the mid and affordable segment, which accounts for most of the shipment volumes in markets such as India.
OEMs such as Motorola and iQOO have shown interest in the new chipsets and are planning to use them in their upcoming smartphones. iQOO said that it's Z6 Lite will be the first smartphone to be powered by Snapdragon 4 Gen 1 chipset.
“Both Snapdragon 6 and Snapdragon 4 provide upgrades in their respective series to enable advancements in the capture, connectivity, entertainment, and AI,” said Deepu John, senior director, of product management at Qualcomm Technologies.

With 5G expected to roll out in more markets including India, these chipsets are expected to play a key role in making 5G accessible to mass buyers. According to Qualcomm, smartphones based on Snapdragon 6 Gen 1 will be available in the January-March quarter of 2023, while those on Snapdragon 4 Gen 1 will hit stores sometime during the July-September quarter of 2022.
Details shared by Qualcomm show that Snapdragon 4 Gen 1 uses Snapdragon X51 5G Modem-RF System and can muster 5G download speeds of up to 2.5Gbps.
Whereas, the Snapdragon 6 Gen 1 uses X62 5G Modem-RF System, which can deliver 5G download speeds of up to 2.9 Gbps and
supports Release 16 of 3GPP (a telco industry standard that enhanced 5G cases).

In terms of performance, Qualcomm claims the Snapdragon 6 Gen 1 can deliver 40% faster processing and 35% faster graphics rendering during gaming. The Snapdragon 4 Gen 1 also offers 15% faster CPU and up to 10% faster GPU performance as compared to its predecessor.
The Snapdragon 6 Gen 1 also includes Qualcomm’s latest AI Engine and is capable of up to 3x better AI performance in terms of intelligent assistance and AI-based activity tracking over its predecessor.
Most mid and high-end chipsets have an AI co-processor to handle AI-related processing. For instance, Google’s Tensor chipset has dedicated AI/ML cores for handling ML-related workloads and reducing the burden on performance cores.
