
Over 90% ML practitioners say their data is marred with noise, bias and gaps
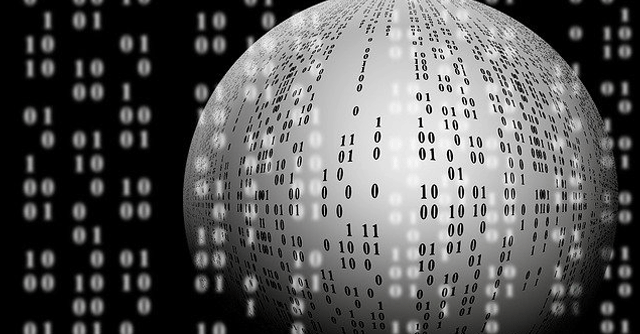

Data quality is one of the most significant tenets on which the success of Machine Learning (ML) practitioners rest. However, according to a recent study by ScaleAI only 9% respondents indicated their data is free from noise, bias, and gaps, which means over 90% ML practitioners say their data is marred with noise, bias, and gaps.
Most (67%) reported that the biggest issue is data noise, and that was followed by data bias (47%) and domain gaps (47%).
Data noise is attributed to meaningless data or a large amount of additional data with unnecessary information.

The study also noted that over one-third (37%) of all respondents do not have the variety of data they need to improve model performance. More specifically, respondents working with unstructured data have the biggest challenge getting the variety of data they need to improve model performance.
The issue is not just not having variety of data but majority of respondents said that they have problems with their training data.
Since a large amount of data generated today is unstructured, it is imperative that teams working in ML develop strategies for managing data quality, particularly for unstructured data.

Also read: Machine Learning can make financial fraud prediction and detection simpler
Also, when it comes to preparing data to train models, respondents cited curating data (33%) and annotation quality (30%) as their top two challenges. The study pointed out that curating data comprises, among other things, removing corrupted data, tagging data with metadata, and identifying what data actually matters for models.
Failure to appropriately curate data ahead of annotation can end up in teams spending time and budget annotating data that is irrelevant or unusable for their models.

Annotating data includes adding context to raw data to enable ML models to generate predictions based on what they learn from the data. Failure to annotate data at high quality often leads to poor model performance, making annotation quality of paramount importance, it said.
The study reveals a key artificial intelligence (AI) readiness trend which says that there is a linear relationship between how quickly teams can deploy new models, how frequently teams retrain existing models, and how long it takes to get annotated data.
Teams that deploy new models fast (especially in less than one month) tend to get their data annotated faster (in less than one week) than those that take longer to deploy. Teams that retrain their existing models more frequently (e.g., daily or weekly) tend to get their annotated data more quickly than teams that retrain monthly, quarterly, or yearly.

However, it is not just the speed of getting annotated data that matters. ML teams must make sure the correct data is selected for annotation and that the annotations themselves are of high quality.
The study notes that aligning all three factors — selecting the right data, annotating at high quality, and annotating quickly takes an intensive effort and an investment in data infrastructure.