
Google research shows why most of the widely spoken languages are not available on the Translate app
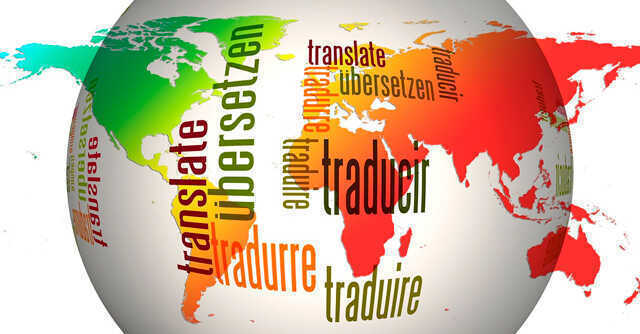

Amid growing internet penetration, online companies such as Google have also stepped-up efforts to support more languages and improve the accuracy of translations. However, there is still a long way to go. Google’s practical machine translation (MT) systems support only a hundred-odd language, which is a tiny fraction of the over 7,000 languages spoken across the world. These systems are also found to be “skewed in favour of European languages,” said researchers at Google in a new research paper titled “Building Machine Translation Systems for the Next Thousand Languages” and published by Cornell University.
“Despite high speaker populations, languages spoken in Africa, South and South-East Asia and indigenous languages of the Americas are relatively under-served,” the research paper stated.
For instance, Google Translate supports lesser spoken languages such as Frisian, Maltese, Icelandic, and Corsican, which as per the research have less than 1 million speakers, but Indian languages such as Bhojpuri, which has over 51 million speakers, or African languages such as Oromo, which has 24 million speakers were not supported at the time of research. Bhojpuri was added to the system in May at the Google I/O 2022 developer conference along with 23 other languages, which have a combined speaking population of 300 million people.

The researchers claim that it was the findings of this research that led Google to address the gap and bring support for 24 new widely spoken languages to Google Translate.
One of the problems that researchers building machine translation systems face is the lack of digitised and accessible datasets and natural language processing (NLP) tools like language identification (LangID) models. In comparison, digitised datasets are more easily available for higher resource languages.
The researchers first built a monolingual web text corpora (dataset) in over 1,500 languages and then scaled LangID models to cover these languages.

In machine translation, a computer program automatically translates text in source language to another language. NLP is a subset of AI that trains machines to understand text and voice and respond to them as humans would.
Using this they built an MT model that can translate over 1,000 languages, utilising Google’s existing corpus used for the 100 odd languages. During the research, native speakers of these languages were consulted and asked to guide and evaluate the development of the MT systems.
“Many languages have a wide variety of dialects, sometimes hardly mutually intelligible. Native speakers helped us understand when our models were producing a particular dialect or mixing and matching them,” the researchers said.

The research paper concluded that using semi-supervised LangID models, document-level consistency signals, and word-based and custom filtering techniques to identify and filter web text in long-tail languages, allowed them to build a multilingual unlabeled text dataset containing over 1 million sentences in more than 200 languages and over 100 thousand sentences in more than 400 languages.
The researchers lamented that errors produced by MT models with zero-resource will persist in the future. However, they believe that it can be addressed by utilizing bilingual dictionaries or similar resources in model translations. They also added that web-mined datasets are a poor alternative to hand-curated datasets and focus on building hand-curated datasets to build text datasets for languages with a limited online presence will lead to more success in making translation work for more global languages.