
‘India has one of the most sophisticated energy management systems at the transmission level’
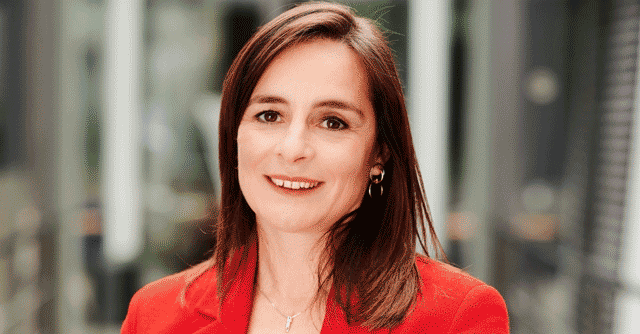

The electric grid is a key piece of infrastructure for countries today. However, with climate change, and the advent of renewables (like wind and solar) and higher demand, the pressure on this 100-year-old technology has increased. Companies like GE Electric are turning to advanced and emerging technologies like artificial intelligence (AI), machine learning (ML) and blockchain to make electric grids smarter. In an interview, Vera Silva, chief technology officer (CTO), Grid Solutions business at GE Renewable Energy, explains how the electric grid is being made more intelligent with the help of these tech tools. Edited excerpts:
How smart are electric grids in India?
The grids in India don’t have enough resiliency—there are interruptions, flickering, etc. — there’s quite a lot of work to be done to improve the power quality of the grids in India.

That’s partly because the infra isn’t keeping pace with the growing demand for electricity. We also don’t have a lot of redundancy in the grid yet because of the infra. The distribution substations and even some transmission substations are ageing and don’t always have the latest technology. There’s a very strong push on costs, which may bring shorter benefits, but can bring additional costs later.
How is the new energy mix of renewables and traditional energy sources impacting grids around the world?
The first one is the way we’re producing energy. Renewables aren’t going to be located where the resources are available, (they will) often be away from the demand centers. Even for the photovoltaic cells (PVs), we have rooftops but we also (have), and especially in the case of India, large PV farms that will be explored. Also, the type of machines that we use to generate aren’t the traditional synchronous machine. The machines are designed to make the most of the wind and the sun.

The second area of change is where the resources are connected. The grid is separated between high-voltage transmission, where we always have flows in two directions, and the distribution networks which go up to our houses, which are designed for one-way flows to go up to our houses. So all the protection and controls assume this type of behaviour. With renewables, a lot of this is distributed, so we’re going to be connecting a large amount of generation into these distribution networks, which weren’t designed for that and aren’t very smart. Hence there’s a huge need for investment, monitoring, observability and orchestration tools on these distribution networks. And the last thing is how people are using electricity. We have a wealth of data and understanding on the behaviour of consumers.
What areas need to be improved to address these issues?
Digitization of the grid is a journey. It’s something that started back in the 60s and 70s with analog, relays, etc. Transmission grids were more advanced by default. For example, India has one of the most sophisticated energy management systems at the transmission level with the largest number of PMUs (Phasor Measurement Units), which are typically sensors that help keep the grid stable.

The area we need the most progress is the distribution grid because it was not designed to host generation, control it or behave and modify the way you regulate (energy). What we’re doing today is getting more observability of distribution through sensors, monitoring, communication, and management systems based on software. There’s also a lot of development in some pockets of Europe in what we call self-healing grids, which reconfigure (automatically) when they notice a fault.
Hence, digital orchestration and automation of the grid are key areas. Last, but not the least, another key area is the protection of the grid since we need to protect equipment and users of the grid.
Can you give us some examples of technologies used in smart grids such as AI, ML and their applications?
The backbones of the smart grid are very much the software that's used to orchestrate the grid — to manage distribution, controls, monitoring, and the data from the grid. All these factors help you to operate (more efficiently). Having more data, information, and visibility opens up new opportunities. That's why AI and ML are critical.
For example, to operate the grid, the better we can forecast the generation and demand to situate the storms, the better we can prepare. What we are doing today is collecting a lot of data. For example, to predict what PV (photovoltaic) is going to be producing, how the demand will behave, etc. This is where combining imaging, deep learning, and other AI techniques is beneficial to make predictions about (things like) distributed energy resource management and wind demand forecasting.

The second is asset performance management. We are adding sensors in every equipment that goes into the grid. Accessing the data from these sensors allows us to be more effective on how we do, for example, more predictive maintenance rather than a time-based one. Last, but not the least, is that on a decentralized grid, you could create islands and micro-grids that could survive and bring electricity faster locally (in case of a grid failure), rather than waiting for the whole grid to be back. This is another area where a lot of these techniques on machine learning can be used. And that's where I talk about the self-healing network.
What role does technology play in predicting electricity usage patterns amid climate change?
Climate variability is a very important area where we work with meteorological models and that’s where high performing computing (HPC) and quantum computing will play a critical role. It's much more complex to forecast at the farm level than it is at the India (country-wide) level. You have cameras that look at cloud movements, and you have new ways of identifying and predicting people’s behaviour. For example, when predicting solar locally, deep learning is unbeatable since we get images of the clouds that are excellent for image processing. And this is completely new for an industry that has always done forecasting based on the time series models.
