
This deep neural network just helped NASA discover 301 exoplanets
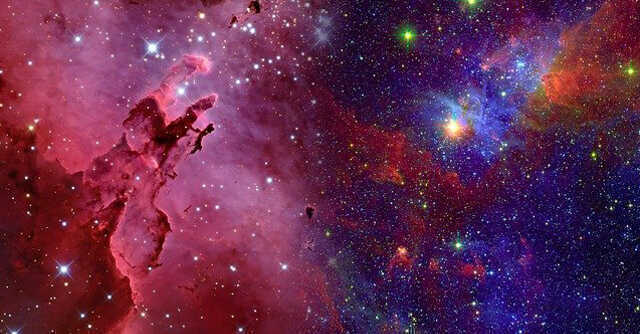

Discovering exoplanets was so far a task that required extensive involvement of human beings, even with the computers’ assistance. But, now NASA has a new member in its exoplanet discovery task force — the ExoMiner deep neural network, which has assisted the scientists confirm discovery of 301 exoplanets spread across distant solar systems.
Exoplanets discovery is a key part of space research and exploration.
An exoplanet is any planet outside our own solar system, which scientists observe using telescopes. They then note down the characteristics exhibited by them, such as the nature of refracted light from the planet(s) in question, to determine whether it is a planet — or some other planetary body such as a satellite straying near a star.

Such discoveries are crucial in the endless quest to understand conditions of life in other solar systems. Programmes to discover exoplanets are designed to help identify potentially habitable worlds outside Earth. However, one tricky area has always been the number of false positives that scientists would identify.
It is this, and all of the above points, that ExoMiner is helping resolve. Jon Jenkins, exoplanet scientist at NASA's Ames Research Center in California, said, “Unlike other exoplanet detecting machine learning programs, ExoMiner isn't a black box—there is no mystery as to why it decides something is a planet or not. We can easily explain which features in the data lead ExoMiner to reject or confirm a planet.”
Hamed Valizadegan, a machine learning manager with the Universities Space Research Association at Ames and the project lead for ExoMiner, said, “When ExoMiner says something is a planet, you can be sure it's a planet. ExoMiner is highly accurate and in some ways more reliable than both existing machine classifiers and the human experts it's meant to emulate because of the biases that come with human labeling.”

The study of using ExoMiner has been published in the Astrophysical Journal. The scientists now claim that this deep neural network model can be applied on other planet discovery setups as well. The latter can increase the efficiency of exoplanet identification, which can then allow scientists to allocate their resources into the more granular aspects of outer world research.