
‘Search provides a very fundamental benefit to society’: Google Search Rankings Head, Pandu Nayak
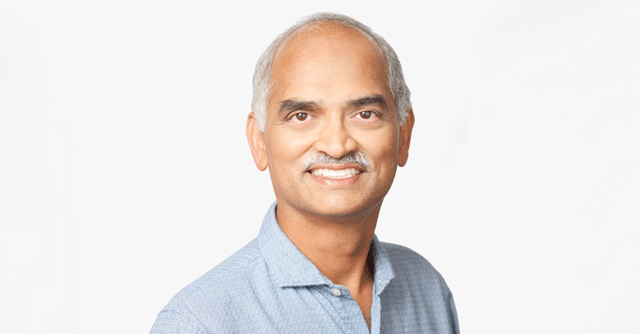

Having worked at Google for more than 16 years, Pandu Nayak, vice president of Search and Google Fellow, leads the company's ranking teams. Nayak was earlier an Adjunct Professor in Stanford University’s Computer Science department. In his earlier roles, he was also a research scientist at the NASA Ames Research Center and served as the deputy lead of the Remote Agent project, which was the first Artificial Intelligence (AI) system to be given primary command of a spacecraft.
A B.Tech. in Computer Science and Engineering from IIT-Bombay, and a Ph.D. in Computer Science from Stanford University, Nayak in an interview spoke about how Google is trying to improve search continuously and make it understand different languages and dialects better. He also spoke about how Google is trying to address algorithmic biases and introduce more transparency in search. Edited excerpts:
How is Google Search coping up with the complexity of languages, especially in India?

Google has had a commitment to solving language problems for Indian users for a long time. Its mission is to organize the world's information and make it universally accessible and useful. Search has been at the heart of this mission for a long time for all people around the world, and particularly in India. There are many things that make Search what it is today — the fact that it's fast, available in many language, etc., But at the heart of it, it's a language-understanding problem. You need to understand what the user is saying in the query. You need to understand what the document means. You need to match them together.
But how accurate are Google’s search results today?
Over the years, we've developed many systems for language understanding both in India and around the world. Consider this query: “Is sole good for kids?” I remember a time when Google searches would throw up many results about shoes for kids when faced with this query. Today, if you do this query, you'll see that most of the results are about fish, even though an occasional result about shoes still creeps in. So, we haven't quite nailed it. This query is on the boundary of what we can do.

Language is subtle. Different languages around the world have their own challenges but in India, there are very special challenges and ones that I'm sort of acutely familiar with because I grew up in India. Like a lot of Indians, I was multilingual. This experience is common to most people. And when you speak, you do not limit yourself to one language--you often mix multiple languages. So, there's another challenge there, not to forget (the hundreds of) dialects.
How are your language models like BERT, MUM and LaMDA assisting in this task?
BERT (Bidirectional Encoder Representations from Transformers) led to one of the largest improvements over the last five years in our ability to understand language and improve Search. (BERT models consider the full context of a word by looking at the words that come before and after it). More recently, we announced a new model called MUM, which stands for Multitask Unified Model. It’s a large model trained on 75 different languages all at one time. The advantage of training it on all these languages simultaneously is that it allows us to generalize from languages. And, by being able to train these languages all at one time, we get to share a sort of underlying structure to generalize these languages with less data.

The other cool thing about MUM is that it is multimodal, which means it can handle both text and images for now, and in the future, videos and audio. LaMDA is a model that was designed with conversation in mind, and it's developed by our research team. It's still a research prototype but an exciting one.
What Search innovations are you introducing for India?
When you issued a query in, let's say Hindi, you would often get results in English. That’s because there isn't as much information on the web in Hindi. So, we take English language content from a set of sites and translate it into Hindi. Now the results are automatically translated into Hindi (or other Indian languages), which makes that English language content accessible to users in their own language.

But even if you get the results in Hindi, there are some users for whom literacy is a bigger challenge. They're more comfortable with the spoken word. So, we have a link on the page that says: “Ise suno” (listen to this). Thus, you can hear the answer. This text to speech is again one of those things that is powered by machine learning. It makes this kind of content even more accessible.
These are just two examples of our sort of longstanding commitment to continue to improve search in India and improve language understanding in these areas.
You have argued that too much transparency of search algorithms can be counterproductive by giving, for instance, spammers more tools to mess around with these algorithms. How do you balance this view with that the equally compelling need for explainable AI, or the need to be transparent about how unsupervised algorithms arrive at decisions?

Let me address the transparency part. A key part of what we do in Search is evaluation, and we put together what we call ‘rater guidelines’. This 170-page document tells raters what Great Search is, which can be sort of distilled down into one sentence -- returning relevant results from reliable, high quality sources whenever possible. This is a public document. Further, the bulk of the search results are not personalized. They are localized to a particular location you are in to provide you with relevant information. For instance, if you’re searching for a South Indian restaurant in Delhi, you do not want to see a list of restaurants in Bangalore or Chennai. Search results are unlike a social feed, which is intrinsically personalized. They are public. So that's another element of transparency.
The point about algorithmic transparency is that it leads to bad outcomes that spammers can start using it to manipulate search results. And when that happens, users don't win because now you'll start getting spam. We go to great pains to protect search results from spammers.
Explainability, too, is sort of a central tenet for Google Search since it was founded. We can't improve our search engine if our engineers don't understand what is happening when they see a flaw in the system. They need to be able to figure out why it happened so that they can develop the next generation of algorithms that addresses the underlying cause. Machine learning systems that we talked about with BERT and others, of course, are less explainable than the systems we used to have, but they're very powerful. We still have a lot of control over our system and the ability to improve it, even as we benefit from powerful new technologies.

What is your sense of where is AI moving, from, for the good of society and what are the kind of balances that we could look at?
I think Search provides a very fundamental benefit to society. And we've been able to harness technology to improve Search. Having said that, technology can be used and be misused. This technology (Search) also raises some challenges around things like bias. You must ensure that you test your systems adequately to try and mitigate these areas of bias. This is a, a big area of emphasis for us.