
How to Boost Training Data for Better Machine Learning
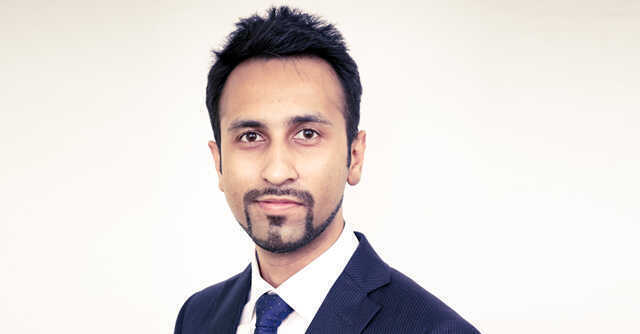

Poor training data is more common than anticipated. Good quality data is a game-changer for machine learning applications. Many projects fail to make it to production due to insufficient or conflicting real-world training data. Knowing beforehand whether there is a sufficient quantity of training data is a big challenge as data scientists can only know that they have enough data when problem-solving succeeds.
There is little guidance from the machine learning (ML) platforms in this situation, and some of the best strategies that can be used to boost training data are not widespread or well-documented.
To tackle some of the shortfalls of training data, many of the below techniques can be followed, either in isolation or combined to improve the quality as well as quantity of training data.

Technique 1 - Expand your data collection strategy.
Current data collection strategies are still geared to satisfy the requirements of the past, which were mainly of a transactional and regulatory nature. Yet, much more data exists inside the enterprise. There are also completely new sensor readings out there that often come at no extra costs. An example being Wi-Fi signatures in public spaces, which can be used to anonymously track pedestrians.
Technique 2 - Spend more time on data pre-processing

There’s no doubt that raw, real-world data can be messy. That’s the reason why data pre-processing takes such a prominent role in ML. For example, removing outliers, finding missing variables, oversampling, etc. Along with these, many data science platforms contain tools and features to support this pre-processing.
Technique 3 - Share data with your peers
Another way to increase the available training data is through data sharing. One of the premier industrial examples of data sharing exists in the context of credit card fraud detection, where the results of ML directly benefit from shared data. Create national or international alliances that collect data together and use federated learning approaches so that data can be shared among enterprises without compromising data privacy.

Technique 4 - Cautiously crowdsource data
Crowdsourcing represents another avenue to obtain data, labels, classifications, extractions, or transcriptions. This time not from professional data providers, but from a pool of internet users. This pool of knowledge workers can be internal, external or a mix. There are dozens of vendors that maintain workflow systems analogous to virtual factories with content assembly lines that allow for worker training and qualification, optimal job allocation, and various sorts of quality assurances.
Technique 5 - Pool data from external data sets

ML derives tremendous value from having data augmented from third-party sources like weather data, traffic data, census data and economic data. One area that benefits significantly is demand forecasting, but also customer behaviour prediction. While there are significant amounts of commercial and open datasets out there, finding them can be quite difficult.
Technique 6 - Augment data using domain-specific transformations
By 2024, 60% of the data used for the development of AI and analytics solutions will be synthetically generated. Synthetically derived data is beneficial, with much less costs and effort than real-world data sampling. The basic idea is to apply a transformation to an instance of real data to generate a synthetic variation. This is highly popular in the area of image recognition, where new image data can be created using transformations like rotation, cropping, blurring, changing colours, obstruction, translation, scaling and noise filtering.

Technique 7 - Generate data through simulations
A more sophisticated way of synthesizing data is to build simulation models that can serve as a proxy or surrogate for the real world. This technique for boosting training data is still very experimental, and only the most advanced teams are using it. Build simulation models that can serve as a proxy or surrogate for the real world. While this technique for boosting training data is still very experimental, only the most advanced teams are using it.
Technique 8 - Use active learning to minimize expensive real-world sampling

One core way of doing so is to show some sample data to the ML system and see how “confident” or “surprised” it is. This way, the ML framework itself is being instrumentalized to choose its next training examples itself
Technique 9 - Use transfer techniques to utilize data that is non accessible
Transfer learning is the reuse of already trained ML models as a starting point for another ML model. The hope is that the second ML process can be either conducted significantly faster, or more accurately, or with much less training data.
This is known to have good applications in the natural language processing (NLP) arena, and also for visual inspection tasks.
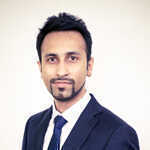
Farhan Choudhary
Farhan Choudhary is a principal analyst at Gartner. Views expressed in this article are his own.