
The role of data engineering and data science in the shipping industry
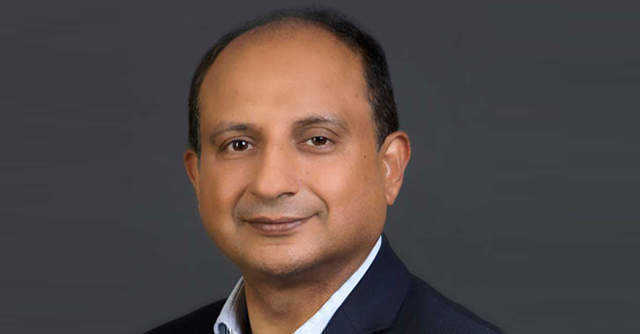

Data is generated, captured, and leveraged during the entire lifecycle of the shipping process. Right from building a client profile, mapping usage and shopping behaviour to delivery, returns, tracking, billing, and end-to-end support. Consider the use case of parcel tracking -- every parcel’s journey has more than 10 events once the order has been placed, these include - pick-up, transit, delay, out for delivery and delivered.
Now consider a company doing thousands of parcel deliveries a day and multiply that by at least 10 events per parcel. This aggregates to a vast amount of data every day for parcel information at just one company. There is plenty of other information for each client, each parcel, and each transaction. And most of this data needs to be stored for long time periods for audit purposes.
Data engineering is the first leg of all the data science algorithms used to derive insights.

With multiple sources of raw data -- carriers, partners, payment service providers, and more -- it is essential to have a refined single source of truth. Data engineering helps create the right data pipeline through ETL (extract, transform, and load), streaming, and event processing, and stores the massaged data in low latency data lakes and warehouses.
To ensure data quality, a crucial aspect of data engineering, companies are utilizing open source frameworks and plug-ins that offer daily checks and use case specific filters, in addition to in-house codes.
Data governance and security, critical pieces especially in global shipping, are also managed through data engineering. One of the biggest use cases in the shipping industry, the real-time chain of custody of a parcel i.e. knowing where the parcel is at a particular time, is also done through data engineering.

It is only after all the data is gathered and cleansed, that it can be used for data science coupled with AI, ML, operation research, and simulation algorithms for business decision-making. Data science is essentially an overlap of programming, technology, business, domain, and statistics. It enables organizations to forecast outcomes to better plan and optimize their top and bottom lines.
There are three broad sets of data science use cases in shipping:
Machine Learning based estimations and predictions
- Volume prediction – Based on historic data and pattern analysis such as on seasonality and trends, data science helps forecast future volumes. Having a view of incoming and outgoing volumes enables business forecasting and better planning of infrastructure and resources, both human and machine.
- Delivery date estimation – Algorithms consider zip codes, real time climate and traffic data, and couple them with historic data to estimate delivery dates for parcels. Accurate estimation of delivery dates improves end user experience. Such algorithms also help companies offer monetizable services such as ‘Guaranteed Delivery’ of packages to clients.
- Return prediction – data Science can help analyze the return potential of a package basis product reviews, quality of service in a zip code, and historic client behavior.

Using forecasts for operational optimization
- Facility management/warehouse management – Operation research methods helps manage facilities better. With a view of potential demand, companies can plan labor, distribution of workload, and prioritize client demand.
- Freight management and fuel efficiency – Real-time data analytics allow route optimization of vehicles and freight for faster deliveries.
Replicating or simulating the real world
Simulation techniques can help replicate the entire network of a shipping firm, from client demand and warehouse operations, to fulfilment, shipping, deliveries, and so on. Simulation gives an end-to-end view of how the network will perform under hypothetical conditions, which parcel journey leg could be a bottleneck or which could cause delays in shipments. For example, a view of a potential high volume of incoming parcels, much above the capacity of parcel facilities or existing workforce, can help companies prepare to action the insights and plan resources accordingly.

In today’s world, one cannot imagine the shipping industry without data. Capturing and leveraging precise and accurate data requires advanced talent and technology. To build strong data engineering and data science teams, it is essential to have the right balance of skills and expertise; mix of senior and junior team members and domain and technology expertise.
Companies must attract young, especially those focusing on applied statistics. Capabilities such as ETL, Kafka, streaming, security and programming languages such as Python must be developed in the workforce.
And finally, domain expertise, a less popular competence but key to data science, must be put on priority.

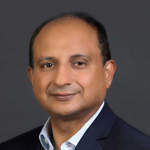
Pankaj Sachdeva
Pankaj Sachdeva is vice president, innovation and India site leader at Pitney Bowes. The views in this article are his own.