
Researchers develop machine-learning algorithm to boost telecom, diagnostics
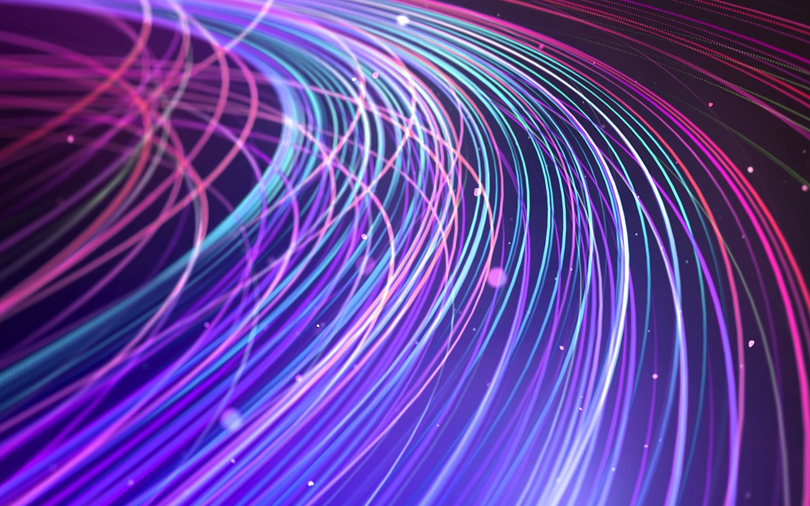

A team of researchers has developed a machine-learning algorithm that can accurately reconstruct images transmitted over optical fibres for distances up to a kilometre, said monthly journal Optica. This approach could improve medical diagnostics and telecommunications.
The journal said that the group of researchers has succeeded in teaching a type of machine-learning algorithm called deep neural network to recognise images of numbers from the pattern of spots they create when transmitted to the far end of a fibre.
This research can boost the supply of information through fibre-optic telecommunication networks, improve endoscopic imaging in medical diagnosis, and increase the optical power delivered by fibres.

"We use modern deep neural network architectures to retrieve the input images from the scrambled output of the fibre," said Demetri Psaltis, Swiss Federal Institute of Technology, Lausanne, who led the research in collaboration with colleague Christophe Moser. "We demonstrate that this is possible for fibres up to one-kilometre long," he added, calling the work "an important milestone”.
The optical fibres, which carry information in the form of light, are multimode fibres with multiple spatial channels to transmit different streams of information simultaneously. The transmission of images, however, has been relatively difficult as light from the images passes through all the channels to create a spotted pattern, which the human eye finds hard to decode.
Psaltis and his team used the deep neural network, a type of machine-learning algorithm that mimics the way a brain functions, to solve this problem.

They had the inputs processed through multiple layers of artificial neurons, each of which conducts a small calculation and passes the result to the next layer. Consequently, the machine learned to identify the input by recognising the patterns of output associated with it.
Elaborating on the similarity of the algorithm to the workings of the brain, a fellow student on the project, Eirini Kakkava, said, "When a person stares at an object, neurons in the brain are activated, indicating recognition of a familiar object. Our brain can do this because it gets trained throughout our life with images or signals of the same category of objects, which changes the strength of the connections between the neurons."
The researchers, to train their system, used a database of 20,000 samples of handwritten numbers, zero through nine. Using 16,000 as training data, they kept aside 2,000 for validation and the remaining 2,000 to test the validation system.

While the spot collected for each digit looked the same to the human eye, the neural network could discern differences and note the intensity pattern associated with each digit.