
Why firms fail to scale AI and how can they solve it
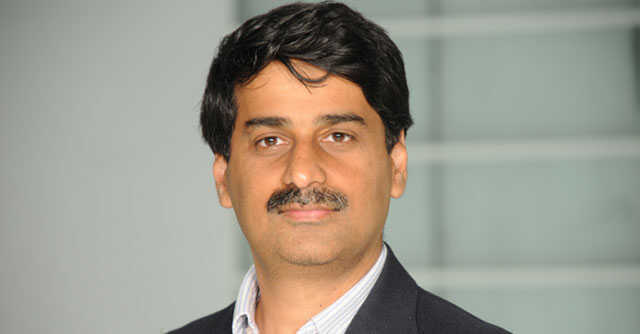

Today, every business wants to invest in AI. Unfortunately, most of them take a product-centric or use case-based approach to adopting it which not only slows down their AI journey but creates additional risks.
An organization may use an off-the-shelf solution or opt for a vendor software for its AI needs. As each use case gets proven, the ambition to scale AI is jeopardized because these initiatives often create technology silos. The software processes used to develop models are inconsistent and the AI models are not shared across teams. Also, many of these products fail to keep up with the fast evolution of AI.
When creating an AI roadmap, organizations must be mindful that the AI engineering life cycle differs from traditional IT Ops/DevOps and is typically more complex. In addition, it is increasingly becoming harder to predict the longevity of fast-evolving AI technologies. Before investing in different AI architectures, organizations must adopt all-encompassing architecture principles and governance to ensure they stay relevant.

The use of AI is still not all-encompassing in many large organizations due to the above-mentioned hurdles. To achieve a mature Enterprise AI, organizations must consider the following three ways to design their architecture.
First of all, organizations need a strong set of governing principles to ensure future AI developments are transparent and connected throughout the organization. A modular approach allows developers to remove or modify AI models that do not serve an organization’s needs.
Layered architecture helps divide the organizational platform into multiple layers, with each layer having its own responsibility. The layering localizes the impact of future changes to a few components within a single layer. The division based on tasks and responsibilities also removes the dependency on any single vendor. Organizations must have the ability to switch between technologies and vendors based on their service quality and price. The AI platform must also allow addition of new capabilities that are vendor-agnostic for faster AI adoption.

Secondly, they can democratize AI through crowdsourcing. AI democratization is the process of widening the user base, which involves participation from all AI stakeholders. AI development requires large resources such as computing power, funds, and expert-level knowledge. AI democratization speeds that up. Even the big-name player Meta recently open sourced its AI translation tool that works across 200 languages, as it clearly saw a limit to doing it alone despite being cash-rich.
Companies should offer a unified vision so that every business function is on the same page. Being transparent about AI metrics, use cases, and interfaces between AI consumers and providers creates opportunities for technology developers to contribute easily.
Data engineers, data scientists, ML engineers, and developers with different skillsets should be allowed to operate independently but encouraged to collaborate to solve common problems. Crowdsourcing tactics like hackathons and competitions to develop new AI assets should be encouraged. Also, by supporting diverse AI tool sets, software development kits, and user channels, one can give more choice to the users and allow different technology groups to participate in AI development.

Thirdly, when working with AI models and in a cloud-first environment, scalability should be a primary consideration. To build a mature Enterprise AI platform, one must ensure flexibility to scale up or down as the use of AI matures.
Every architectural layer of the Enterprise AI model should be cloud-native to ensure quicker microservices-based architecture deployment and scaling. This allows easy scalability of technology components, higher efficiency, and faster performance.
Here, trust is critical when taking AI enterprise-wide. This can be done by publishing the explanations of AI decision-making logic along with data history and accuracy metrics. Being transparent about your AI decision-making process eases regulatory compliance.

Finally, the use of agile and iterative methodologies is needed for experimentation and proofs of concepts for AI development and deployment. This allows rapid changes during development and easy onboarding of new AI technology.
Focusing on a multi-layered architecture, AI democratization and scalability and their associated principles will ensure that enterprise AI is relevant to a company’s business goals and plays a role in its success.
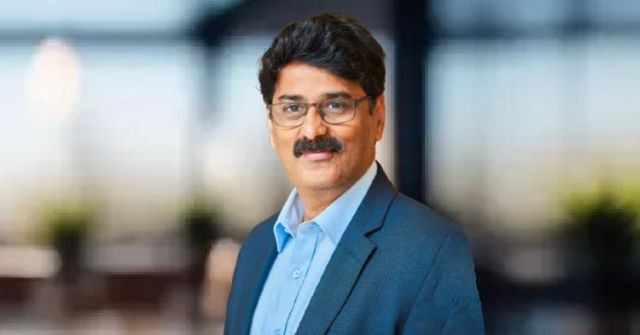
Balakrishna D.R.
Balakrishna D. R is Executive Vice President, Global Services Head, AI and Industry Verticals, Infosys